Enhancing Software Engineering Agents via Scaling Test-Time Compute
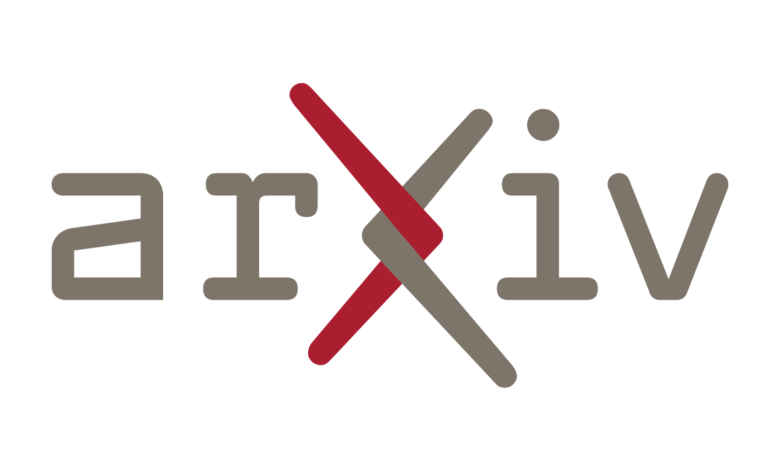
View the PDF file for the paper entitled Thinking for a longer period, and not greater: enhancing software engineering factors by calculating time test time, by Yingwei MA and 7 other authors
PDF HTML (experimental) view
a summary:Recent developments in software engineering agents have shown promising capabilities to automate program improvements. However, their dependence on closed or intense resource models provides major challenges to publishing in private environments, which drives an important question: \ Textit {How can the Open-Open Open Modern Aduled performance?}}
To achieve this purpose, we suggest a unified framework to calculate the test time time test that benefits from increasing the reckoning time instead of the larger models. Our framework includes two complementary strategies: internal TTC and external TTC. Internally, we offer the {Development-ENTEXTualIZED {method {Development-CONTEXTualIZED} way that benefits from the real world programs warehouses to pave multiple stages, such as localization of faults and generation of correction. We increase the promotion of the path of the path by taking rejection samples, and evaluating strict paths along the accuracy and complexity. Externally, we suggest a new {search-process based on the development process} that guides the reward models and verification of implementation. This approach provides the target arithmetic allocation at critical development decision points, and to overcome restrictions on the “final point only” verification methods.
The evaluation assessments of Swe-Bench that are verified \ Textbf {32B Vervision 46 % \ %}, exceeding significantly larger models such as Deepseek R1 671b and Openai O1. In addition, we offer the experimental verification of the phenomenon of scaling the test time within SWE factors, and we reveal that \ Textbf {models customize more distinctive symbols for increasingly difficult problems}, which effectively enhances thinking capabilities. We publicly publish all training data, models and symbols to facilitate future research. This URL https address
The application date
From: yingwei ma [view email]
[v1]
Mon, 31 Mar 2025 07:31:32 UTC (2,462 KB)
[v2]
Tuesday, April 8, 2025 12:36:08 UTC (2,462 KB)
Don’t miss more hot News like this! AI/" target="_blank" rel="noopener">Click here to discover the latest in AI news!
2025-04-09 04:00:00