DeepMind’s latest research at NeurIPS 2022
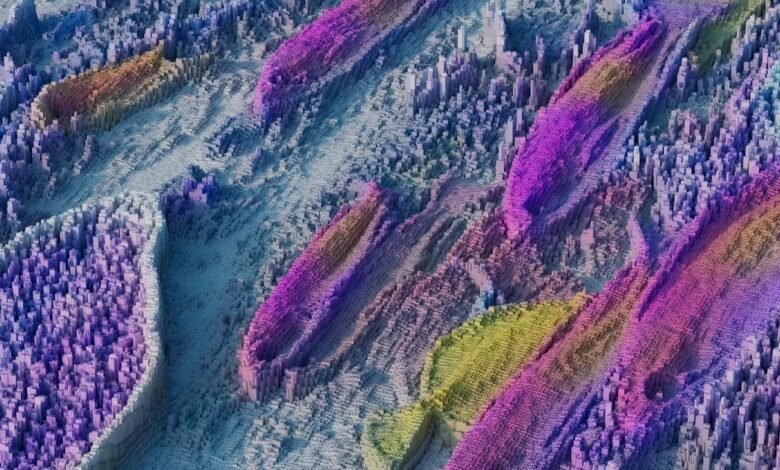
Big models offer the best in their class, improved RL factors, and the most transparent, ethical, and fair systems
The thirty -sixth International Conference on NeuPS 2022 (NEUPS 2022) will be held from November 28 – December 9, 2022, as a hybrid event, based in New Orleans, USA.
Neurips is the largest conference in the world in artificial intelligence (AI) and machine learning (ML), and we are proud to support the event as diamond sponsors, which helps to enhance the exchange of research progress in the AI and ML community.
Deepmind teams offer 47 sheets, including 35 external collaborators in virtual paintings and sticker sessions. Here is a brief introduction to some of the research we provide:
Big models are the best in their class
Grand models (LMS) – Trucitomic IQ trained on huge amounts of data – resulted in incredible offers in fields including language, text, sound and images generation. Part of their success is due to their huge scope.
However, in Chinchilla, we created a 70 billion parameter language model that exceeds many largest models, including GoPher. We updated the scaling laws of the large models, which show how the previously trained models were very large for the amount of training that was conducted. This work has already constituted other models that follow these updated rules, create smaller and better models, and won the distinguished main path paper award at the conference.
Based on Chinchilla, our multimeds and Perceiver, we also offer Flamingo, a family of the few visual language models that learn. Flamingo represents photo processing, videos and text data a bridge between only vision models and language. The Flamingo model places one on the latest learning model a few shots on a wide range of open media tasks.
However, the size and architecture are not the only important factors for the power of transformers. Data features also play an important role, which we discuss in a presentation on data features that promote learning within context in transformer models.
Improving reinforcement learning
Reinforce learning (RL) showed a great promise as a approach to creating generalized AI systems that can deal with a wide range of complex tasks. This has led to breakthroughs in many areas from moving to mathematics, and we are always looking for ways to make RL agents more intelligent and smaller.
We present a new approach that enhances the decision -making capabilities of RL agents in an effective manner by expanding the dramatically available information for its retrieval.
We will also show a simple, conceptual approach to curiosity based on visually complex environments-a RL factor called Byol-EXPLORE. It achieves a superior performance while it is strong for noise and being much simpler than pre -work.
Al -Khwarizmi progress
From data pressure to run to weather forecasting, algorithms are an essential part of modern computing. Thus, additional improvements can have a tremendous effect when working widely, helping energy, time and money.
We share a new and highly developed new method for automatic composition of computer networks, based on the algorithm thinking, which indicates that our very flexible approach reaches 490 times faster than the current state of art, while meeting the majority of input restrictions.
During the same session, we also offer a strict exploration of the previous theoretical concept, “The algorithm”, while highlighting the accurate relationship between nerve networks and dynamic programming, and the best way to integrate it to improve the performance of external distribution.
The leading responsibility
At the heart of the Deepmind mission, our commitment to acting as officials in the field of artificial intelligence. We are committed to the development of artificial intelligence systems, transparency, ethical, and fair.
Explanation and understanding of complex artificial intelligence systems is an essential part of the creation of fair, transparent and accurate systems. We offer a group of desidata that captures these aspirations, and half a practical way to meet them, which includes training the artificial intelligence system to build a causal model for itself, allowing it to explain its behavior in a purposeful way.
To act safely and morally in the world, artificial intelligence agents must be able to think about harm and avoid harmful measures. We will present a cooperative work on a new statistical scale called anti -damage damage, and we explain how it overcomes problems with standard methods to avoid following harmful policies.
Finally, we offer our new paper that suggests ways to diagnose failure and mitigate this in the typical integrity caused by distribution attacks, which indicates the importance of these problems to spread safe ML technologies in healthcare places.
See the full range of our work in Neurips 2022 here.
Don’t miss more hot News like this! Click here to discover the latest in AI news!
2022-11-25 00:00:00