Former DeepSeeker and collaborators release new method for training reliable AI agents: RAGEN
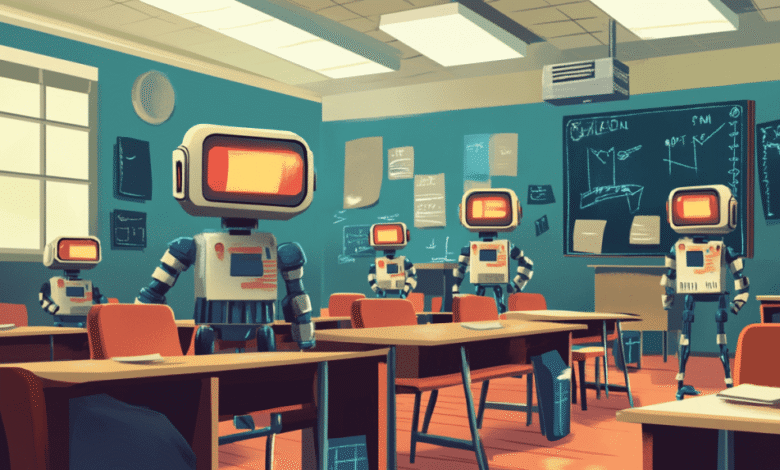
Join daily and weekly newsletters to obtain the latest updates and exclusive content to cover the leading artificial intelligence in the industry. Learn more
It was supposed to be in 2025, through many accounts of experts, the general artificial intelligence agents-AI applications for the task supported by leading linguistic models and large benefits (LLMS) such as the species provided by Openai, anthropic, Google and Deepseek.
But so far, most of the artificial intelligence agents are still stuck as experimental spectrums in a type of disinfectant for companies, according to a recent survey on the social network X.
Road assistance may be: a cooperative team from North Westin University, Microsoft, Stanford, and Washington University-including a former diplomatic researcher named Zihan Wang, who is currently completing a PhD in computer science at Northwestern- introduces Ragen, a new system of training and evaluation of AI customers more reliable and less free.
Contrary to fixed tasks such as mathematics solution or code generation, Ragen focuses on multi -turn interactive settings where agents must adapt, remember, and mind in the face of uncertainty.
It was built on a dedicated RL frame called StarPo (Politics Improvement-Intellectual Acts), the system explores how LLMS can learn through experience instead of memorizing. The focus is on the entire decision -making paths, not just one step response.
Starpo works in two tangled phases: the deportation stage where LLM creates complete reaction serials that are guided by thinking, and an update phase where the model is improved using natural cumulative rewards. This structure supports a more stable and interpretative educational seminar compared to the methods of improving standard policy.
The authors have applied and tested the frame using alibaba QWAN models, including QWEN 1.5 and QWEN 2.5. These models were the basic LLMS of all experiments and were chosen for their open weights and strong capabilities to follow the instructions. This decision enabled reproduction and basic comparisons fixed through symbolic tasks.
Here is how they did that and what they found:
Echo trap: How to reinforce learning bonuses lead to LLM thinking
Wang summarized the basic challenge on the common x theme on a large scale: Why are your RL training always collapsed?
According to the team, LLM agents initially create symbolic responses and well. But with the passage of time, RL tends to reward the shortcuts, which leads to frequent behaviors that put out of general performance – a pattern they call the “Echo trap”.
This slope is driven by counter -feeding rings, as some phrases or strategies gain high bonuses early, and to encourage excessive use and suffocating exploration.
Wang notes that the symptoms are measurable: slopes in the variation of rewards, gradient nails, and the effects of thinking disappear.
Ragen test environments are not exactly at the level of the institution
To study these behaviors in a controlled environment, Ragen evaluates factors across three symbolic environments:
- Thieves: One task of randomness that tests symbolic thinking of risk reproduction.
- SukobanMulti -turning puzzle, which includes irreversible decisions.
- Frozen: Stoxic mission, multiple turns that require adaptive planning.
Each environment is designed to reduce young people in the real world and focus only on decision -making strategies developed during training.
In the thieves environment, for example, the agents are informed that the arms of Dragon and Phoenix represent different rewards distributions.
Instead of being informed of the possibilities directly, they must think symbolically – EG, and the interpretation of Dragon as “power” and phenyx as “hope” – to predict the results. This type of preparation presses the model to generate interpretable analog thinking.
Reinforce learning with StarPo-S
To address the collapse of the training, researchers presented Starpo-S, a stable version of the original frame. Starpo-S includes three main interventions:
- Liquidation of decline based on uncertainty: Giving priority to a proposal where the agent shows uncertainty in the results.
- KL penalty removalAllow the model to deviate freely from its original policy and explore new behaviors.
- PPO scraps is asymmetric: More high -reward paths than low -bonus paths to enhance learning.
These changes are delaying or eliminating the collapse of training and improving performance in all three tasks. Wang said: “Starpo-S … works in all three tasks. It reduces collapse. Better reward.”
What makes the AI AI model good?
The success of the RL training is not only on architecture, but on the quality of data resulting from the agents themselves. The team selects three dimensions that greatly affect the training:
- Task diversityExposing the model to a wide range of initial scenarios improves generalization.
- ReactionAllow multiple procedures for each role that provides the most meaningful planning.
- FreshnessMaintaining training data in line with the current model policy avoids old learning signals.
Together, these factors make the training process more stable and effective.
An interactive experimental location that researchers published on GitHub makes this explicit pass and visualization to photograph the full dialogue-including not only the procedures, but the process of thinking is step-by-step.
For example, when solving the math problem, the agent may first “think” to isolate a variable, then send an answer like “x = 5”. These intermediate ideas are visible and can be tracked, adding transparency to how agents reach decisions.
When logic is running out
Although frank thinking improves performance in simple and unique tasks such as Bandit, it tends to decompose during multimed training. Although structured claims and symbols are used, the effects of thinking often shrink or disappear unless they are directly rewarded.
This indicates restrictions on how to design rewards usually: focus on completing the task may neglect the quality of the process behind it. The team has tried the penalties based on coordination to encourage organized thinking better, but it admits that there is a need for further formation of the bonus.
Ragen is now available, along with StarPo and StarPo-S business, as an open source project on https://github.com/ragen-ai/ragen. However, a clear license is not listed in the GitHub warehouse at the time of writing this report, which may reduce use or redistribution by others.
The system provides a valuable basis for those interested in developing artificial intelligence agents who perform more than full tasks – think, plan and develop.
As AI continues to move towards autonomy, projects such as Ragen help shed light on what is necessary to train models that not only learn from data, but of the consequences of their actions.
Distinguished questions to adopt the real world
While the Ragen paper offers a detailed technical road map, many practical questions for those who look forward to applying these methods in institution settings remain. For example, how much RAGEN approach is far from symbolic tasks? Do companies need to design completely new and rewarding environments to use this system in the workflow such as processing the bill or customer support?
Another critical field is the ability to expand. Even with the improvements provided by StarPo-S, the paper acknowledges that training is still collapsing on longer horizons. This raises the question: Is there a theoretical or practical path to keep thinking about the sequence of open or evolving tasks constantly?
At the time of writing this report, no explicit license has been listed in a warehouse or documents Ragen GitHub, leaving open questions about use rights.
To explore these and other questions-how should the non-technical decision makers explain the effects of Rajen-I contacted the co-author Wang for more insight. At the time of writing this report, the response is suspended. If any comments arrive, it will be included in a follow -up to this article or merged as an update.
Ragen stands out not only as a technical contribution but as a conceptual step towards the most independent artificial intelligence factors and the ability to think. Whether it becomes part of the AI Enterprise staple, but its visions in the dynamics of the agent’s learning already help redefine LLM training limits.
Don’t miss more hot News like this! Click here to discover the latest in Technology news!
2025-04-23 20:04:00