Researchers from Sea AI Lab, UCAS, NUS, and SJTU Introduce FlowReasoner: a Query-Level Meta-Agent for Personalized System Generation
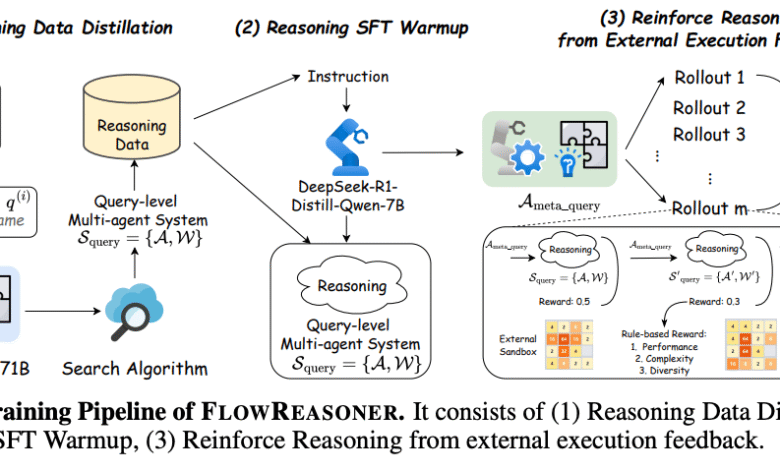
The multi -agent -based systems of LLM that are characterized by planning, thinking, using tools and memory capabilities are the basis of applications such as Chatbots, code generation, mathematics and robots. However, these systems face great challenges as they are manually designed, which leads to high costs of human resources and limited expansion. The chart -based methods tried to automate workflow designs by formulating workflow tasks as networks, but their structural complexity restricts expansion. Modern methods are multi -agent systems such as programming code and the use of advanced LLMS as adequacy to improve workflow, but focus on solutions on the level of task that generates single task systems. This approach that suits everyone lacks the ability to automatically adapting to individual user information.
The multi -LLM dealer systems are the basis for various applications in the real world, including the intelligence of software instructions, computer use and deep research. These systems are characterized by LLM agents equipped with planning capabilities, access to the database, and calling the function of the tool that cooperates to achieve promising performance. Early methods have focused on improving claims or high standards through the development algorithms of stereotypes. ADAS presented the representation of the code for agents and the workflow with the twinning agent to create the workflow. Moreover, Openai has advanced thinking in LLMS by developing the O1 model. She followed models such as QWQ, QVQ, Deepseek, and Kimi, and the development of similar thinking structures O1. The O3 Openai model achieves promising results on the ARG-AGI standard.
Researchers from the SEA AI, Singapore, Chinese Science Academy, National University of Singapore, and the University of Shanghai Jiao Tong, Flowreasoner, have suggested a level agent at the query level designed to create multi -performance systems at the query level, generating a single customized system by user. The researchers distilled Deepseek R1 to supply Flowreasoner with the basic thinking capabilities needed to create multi -agent systems, then strengthened them through reinforcement learning with external implementation reactions. A multi -purpose reward mechanism has been developed to improve training through three critical dimensions: performance, complexity and efficiency. This enables Flowreasoner to create multi -agent personal systems through deliberative thinking for every unique query.
Researchers choose three data collections: Bigcodebench for engineering tasks, Humaneval, and MBPP for algorithm challenges for detailed evaluation through various code generation scenarios. Flowreasoner is evaluated for three categories of basic lines:
- One -style direct protest using independent LLMS
- Manual Workflow tasks include self-decline, LLM-DEBATE, and LLM-Blende
- Improving workflow methods such as AFLOW, Adas and Maas that build workflow through research or improvement.
The O1-MINI and GPT-4O-MINI are used as models for manually designed workflow. Flowreasoner is performed with two Deepseek-R1-Distill-Swen (7B and 14B parameters) using O1-Mini as a worker model.
Flowreason-14B is outperforming all competing methods, achieving a total improvement of 5 percentage points compared to the strongest baseline, Maas. It exceeds the performance of the basic workers model, O1-MINI, with a large margin of 10 %. These results show the effectiveness of the framework of action -based thinking in enhancing the accuracy of the generation of the code. To assess generalization capabilities, experiments are performed to replace O1-MINI models such as QWEN2.5-Claude, Claude and GPT-4O-MINI, while maintaining the level agent either either Flowreason-7B or Flowerium-14B. Flowreasoner presents a noticeable transfer capacity, while maintaining fixed performance through various workers ’models in the same tasks.
In this paper, researchers provide Flowreasoner, a mobilization factor at the query level designed to automate the creation of multiple agents for individual user information. Flowreasoner uses external implementation notes and reinforcement learning with multi -purpose rewards with a focus on performance, complexity and efficiency to create improved workflow tasks without relying on complex research algorithms or carefully designed research groups. This approach reduces human resource costs while enhancing expansion by enabling multiple agents more air -conditioned and effectively that improves its structure based on the specific user ketches instead of relying on fixed workflows for the entire tasks categories.
verify Paper and Jabbab page. Also, do not forget to follow us twitter And join us Telegram channel and LinkedIn GrOup. Don’t forget to join 90k+ ml subreddit.
🔥 [Register Now] The virtual Minicon Conference on Agency AI: Free Registration + attendance Certificate + 4 hours short (May 21, 9 am- Pacific time)

Sajjad Ansari is in the last year of the first university stage of Iit khargpur. As enthusiastic about technology, it turns into the practical applications of Amnesty International with a focus on understanding the impact of artificial intelligence techniques and their effects in the real world. It aims to clarify the concepts of complex artificial intelligence in a clear and accessible way.
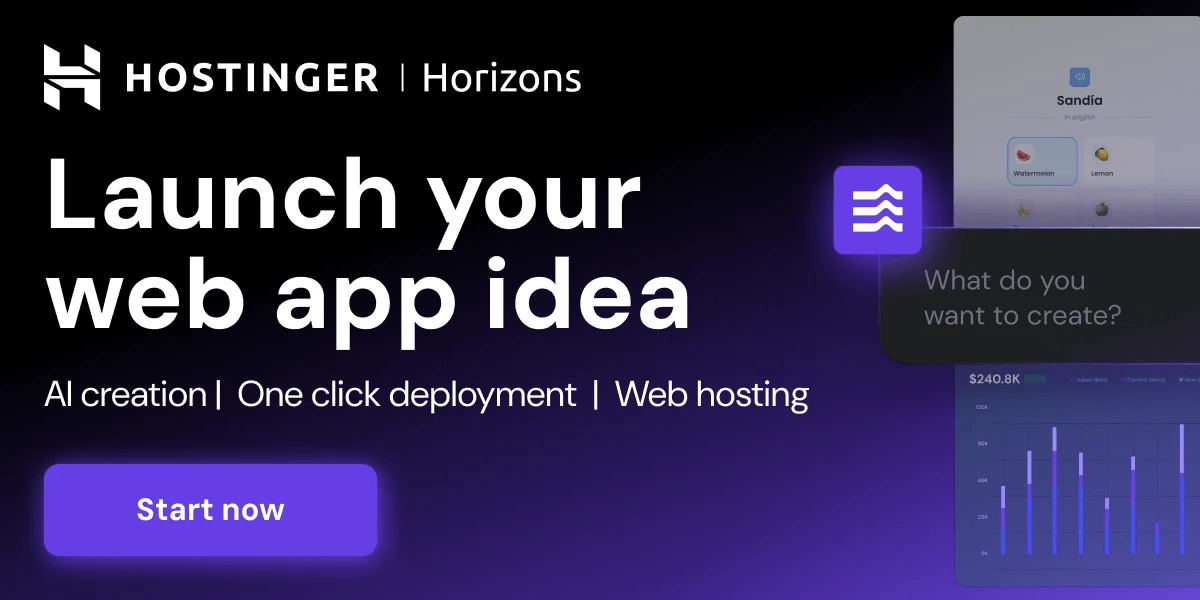
Don’t miss more hot News like this! Click here to discover the latest in AI news!
2025-04-27 20:28:00