A Coding Guide to Different Function Calling Methods to Create Real-Time, Tool-Enabled Conversational AI Agents
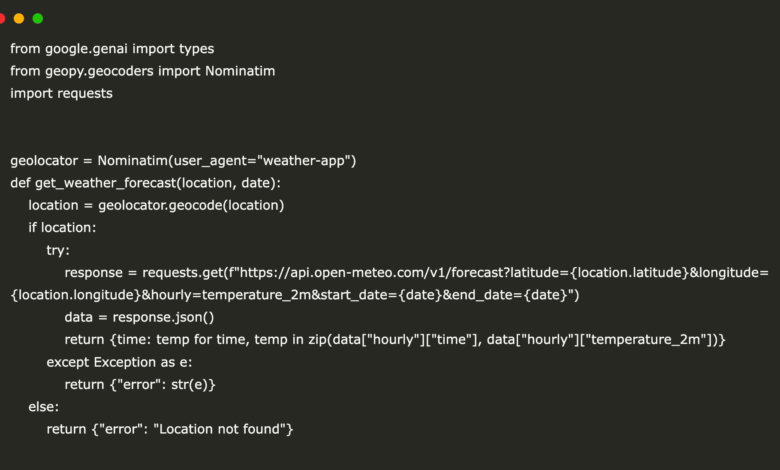
The LLM job is called as a bridge between natural language claims, real world code or application programming facades. Instead of just creating a text, the model decides when a pre -defined job is called, and the JSON call is launched with the name and media name, then waiting for your application to implement this call and restore results. This episode can be underdeveloped, and it may require multiple functions in the sequence, which allows rich multi -step reactions fully under the conversation control. In this tutorial, we will implement the weather assistant with Gemini 2.0 Flash to show how to prepare and manage this communication cycle. We will implement various variables of the job invitation. By combining job calls, we convert the chat interface into a dynamic tool for tasks in the actual time, whether it brings live weather data, verification of demand cases, scheduling dates, or updating databases. Users no longer fill complex models or navigate in multiple screens; They simply describe what they need, and LLM regulates basic procedures smoothly. The natural language automation allows this ease to build artificial intelligence factors that can access external data sources, make transactions or operate the workflow, all in one conversation.
Contact function with Google Gemini 2.0 Flash
!pip install "google-genai>=1.0.0" geopy requests
We install Gemini Python SDK (Google-Genai ≥ 1.0.0), along with Geopy to convert websites to the coordinates and requests of HTTP calls, and ensure all the basic dependencies of our weather auxiliary in Colab.
import os
from google import genai
GEMINI_API_KEY = "Use_Your_API_Key"
client = genai.Client(api_key=GEMINI_API_KEY)
model_id = "gemini-2.0-flash"
We import Gemini SDK, adjust your API key, create an example of Genai.client formed to use the “Gemini-2.0-Flash” model, and create the basis for all applications for subsequent jobs.
res = client.models.generate_content(
model=model_id,
contents=["Tell me 1 good fact about Nuremberg."]
)
print(res.text)
We send the user router (“Tell me 1 good truth about Nuremberg.”) To the GEINI 2.0 Flash model via center_content, then print the text response to the model, showing a basic text call and an end to the tip using the SDK.
Contact function with the JSON chart
weather_function = {
"name": "get_weather_forecast",
"description": "Retrieves the weather using Open-Meteo API for a given location (city) and a date (yyyy-mm-dd). Returns a list dictionary with the time and temperature for each hour.",
"parameters": {
"type": "object",
"properties": {
"location": {
"type": "string",
"description": "The city and state, e.g., San Francisco, CA"
},
"date": {
"type": "string",
"description": "the forecasting date for when to get the weather format (yyyy-mm-dd)"
}
},
"required": ["location","date"]
}
}
Here, we define the jet_weather_forecast chart, determining its name, and descriptive demand to direct Gemini to the time of its use, and the exact entry parameters (location and history) with their types, descriptions and fields required, and thus the model can emit good jobs calls.
from google.genai.types import GenerateContentConfig
config = GenerateContentConfig(
system_instruction="You are a helpful assistant that use tools to access and retrieve information from a weather API. Today is 2025-03-04.",
tools=[{"function_declarations": [weather_function]}],
)
We create GenerateCONTCONFIG tells Gemini that he works as a Retrival assistant and records your weather function under the tools. Thus, the model defines how to create organized calls when ordering expected data.
response = client.models.generate_content(
model=model_id,
contents="Whats the weather in Berlin today?"
)
print(response.text)
This call sends the naked router (“What is the weather in Berlin today?”) Without including the composition (and therefore there are no functional definitions), so Gemini is due to completing the normal text, and provides general advice instead of calling your weather tool.
response = client.models.generate_content(
model=model_id,
config=config,
contents="Whats the weather in Berlin today?"
)
for part in response.candidates[0].content.parts:
print(part.function_call)
By passing the composition (which includes the Json -Schema), Gemini recognizes that Get_weather_Forecast must be called instead of responding in an ordinary text. The episode on the response.[0]CONTENT.PARTS and then print a.
from google.genai import types
from geopy.geocoders import Nominatim
import requests
geolocator = Nominatim(user_agent="weather-app")
def get_weather_forecast(location, date):
location = geolocator.geocode(location)
if location:
try:
response = requests.get(f"https://api.open-meteo.com/v1/forecast?latitude={location.latitude}&longitude={location.longitude}&hourly=temperature_2m&start_date={date}&end_date={date}")
data = response.json()
return {time: temp for time, temp in zip(data["hourly"]["time"], data["hourly"]["temperature_2m"])}
except Exception as e:
return {"error": str(e)}
else:
return {"error": "Location not found"}
functions = {
"get_weather_forecast": get_weather_forecast
}
def call_function(function_name, **kwargs):
return functions[function_name](**kwargs)
def function_call_loop(prompt):
contents = [types.Content(role="user", parts=[types.Part(text=prompt)])]
response = client.models.generate_content(
model=model_id,
config=config,
contents=contents
)
for part in response.candidates[0].content.parts:
contents.append(types.Content(role="model", parts=[part]))
if part.function_call:
print("Tool call detected")
function_call = part.function_call
print(f"Calling tool: {function_call.name} with args: {function_call.args}")
tool_result = call_function(function_call.name, **function_call.args)
function_response_part = types.Part.from_function_response(
name=function_call.name,
response={"result": tool_result},
)
contents.append(types.Content(role="user", parts=[function_response_part]))
print(f"Calling LLM with tool results")
func_gen_response = client.models.generate_content(
model=model_id, config=config, contents=contents
)
contents.append(types.Content(role="model", parts=[func_gen_response]))
return contents[-1].parts[0].text.strip()
result = function_call_loop("Whats the weather in Berlin today?")
print(result)
We implement the full “Agentic” episode: It sends your demands to Gemini, checks the response to the job call, executes Get_weather_Forecast (using Geopy as well as an open HTTP request), then nourishes the tool’s result again in the form to produce and reply to the final conversation.
Call the job using Python functions
from geopy.geocoders import Nominatim
import requests
geolocator = Nominatim(user_agent="weather-app")
def get_weather_forecast(location: str, date: str) -> str:
"""
Retrieves the weather using Open-Meteo API for a given location (city) and a date (yyyy-mm-dd). Returns a list dictionary with the time and temperature for each hour."
Args:
location (str): The city and state, e.g., San Francisco, CA
date (str): The forecasting date for when to get the weather format (yyyy-mm-dd)
Returns:
Dict[str, float]: A dictionary with the time as key and the temperature as value
"""
location = geolocator.geocode(location)
if location:
try:
response = requests.get(f"https://api.open-meteo.com/v1/forecast?latitude={location.latitude}&longitude={location.longitude}&hourly=temperature_2m&start_date={date}&end_date={date}")
data = response.json()
return {time: temp for time, temp in zip(data["hourly"]["time"], data["hourly"]["temperature_2m"])}
except Exception as e:
return {"error": str(e)}
else:
return {"error": "Location not found"}
Get_weather_Forecast first is used from Geopy to convert the city and state chain into coordinates, then sends a HTTP request to the Mteo Open Applications to recover the hourly temperature data for the specified date, and re -dictionary that makes every time to the corresponding temperature. It also deals with errors safely, re -error message if the site is not found or the API is not failed.
from google.genai.types import GenerateContentConfig
config = GenerateContentConfig(
system_instruction="You are a helpful assistant that can help with weather related questions. Today is 2025-03-04.", # to give the LLM context on the current date.
tools=[get_weather_forecast],
automatic_function_calling={"disable": True}
)
This configuration records the Python Get_weather_Forecast function as a communication tool. He sets a clear system mentor (including date) for the context, with the disruption of “automatic_function_calling” so that Gemini sends a job call to the job instead of invoking it internally.
r = client.models.generate_content(
model=model_id,
config=config,
contents="Whats the weather in Berlin today?"
)
for part in r.candidates[0].content.parts:
print(part.function_call)
By sending the claim to use your assigned configuration (including the Python tool but with the disruption of automatic calls), this excerpt picks the Gemini Raw -Call decision. Then fly on each part in response to the printing of an .function_Call object, allowing you to check the tool that exactly wants the model with what the mediators are.
from google.genai.types import GenerateContentConfig
config = GenerateContentConfig(
system_instruction="You are a helpful assistant that use tools to access and retrieve information from a weather API. Today is 2025-03-04.", # to give the LLM context on the current date.
tools=[get_weather_forecast],
)
r = client.models.generate_content(
model=model_id,
config=config,
contents="Whats the weather in Berlin today?"
)
print(r.text)
Through this composition (which includes the fun_weather_forecast function and leaving the automatic virtual calls), calling the conner_content will call GEMINI your weather tool behind the scenes and then return the normal language. Print R.Text outputs, which is a final response, including the actual temperature expectations for Berlin on the specified date.
from google.genai.types import GenerateContentConfig
config = GenerateContentConfig(
system_instruction="You are a helpful assistant that use tools to access and retrieve information from a weather API.",
tools=[get_weather_forecast],
)
prompt = f"""
Today is 2025-03-04. You are chatting with Andrew, you have access to more information about him.
User Context:
- name: Andrew
- location: Nuremberg
User: Can i wear a T-shirt later today?"""
r = client.models.generate_content(
model=model_id,
config=config,
contents=prompt
)
print(r.text)
We expand your assistant in personal context, tell the name Gemini Andrew and its location (Nurmberg) and ask him if the ritual of the shirt, while continuing to use the Get_weather_Forecast tool under the cover. Then it prints the natural language recommendation for the model based on the actual expectations of that day.
In conclusion, we now know how to define functions (via the JSON chart or Python signatures), Gemini 2.0 Flash composition to detect and remove job calls, and implement the “Agentic” episode that implement these calls and consists of the final response. Through these construction blocks, we can extend any LLM to an assistant who is able to connect tools that automate the workflow, recover live data, interact with the code or your application programming facades easily such as chatting with a colleague.
Here is Clap notebook. Also, do not forget to follow us twitter And join us Telegram channel and LinkedIn GrOup. Don’t forget to join 90k+ ml subreddit.
🔥 [Register Now] The virtual Minicon Conference on Agency AI: Free Registration + attendance Certificate + 4 hours short (May 21, 9 am- Pacific time)
Asif Razzaq is the CEO of Marktechpost Media Inc .. As a pioneer and vision engineer, ASIF is committed to harnessing the potential of artificial intelligence for social goodness. His last endeavor is to launch the artificial intelligence platform, Marktechpost, which highlights its in -depth coverage of machine learning and deep learning news, which is technically sound and can be easily understood by a wide audience. The platform is proud of more than 2 million monthly views, which shows its popularity among the masses.
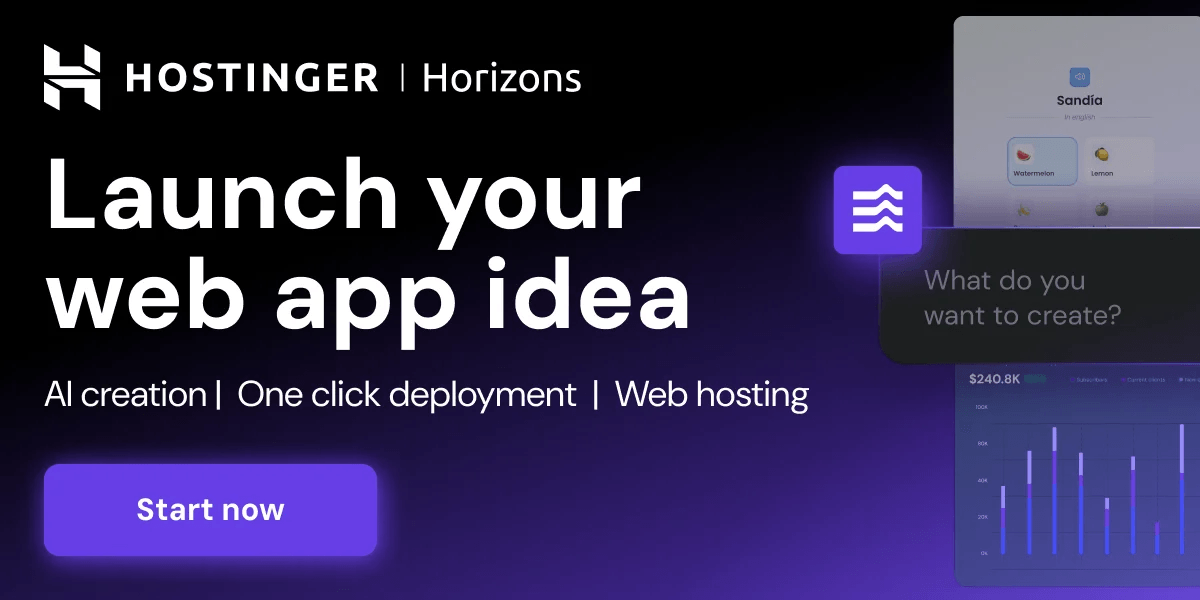
Don’t miss more hot News like this! Click here to discover the latest in AI news!
2025-04-29 07:03:00