Bigger isn’t always better: Examining the business case for multi-million token LLMs
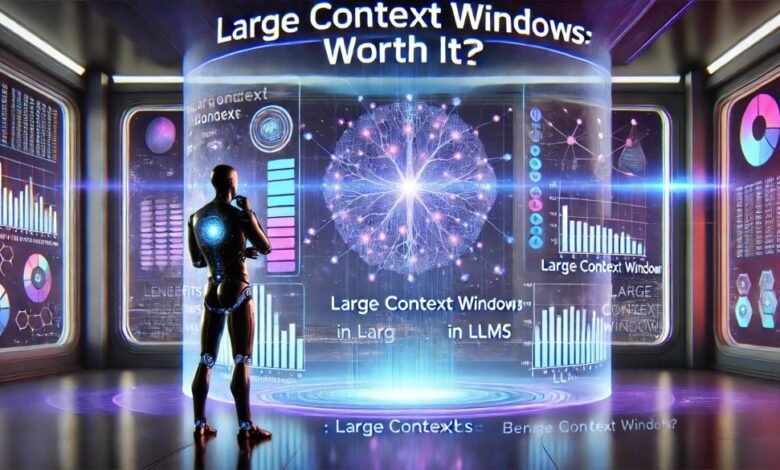
Join daily and weekly newsletters to obtain the latest updates and exclusive content to cover the leading artificial intelligence in the industry. Learn more
The race to expand the large language models (LLMS) exceeds the threshold of millions has sparked a violent discussion in the artificial intelligence community. Models such as Minimax-Text 01 have a capacity of 4 million, and Gemini 1.5 Pro can process up to 2 million icons at one time. They are now promising to change applications and can analyze a full code code, legal contracts or research papers in one conclusion call.
At the heart of this discussion is the length of context – the amount of the text that the artificial intelligence model can treat as well Remember Once. The tallest context window allows the ML to deal with more information in one request and reduces the need to install documents in sub -arguments or divide the conversations. For context, the model with a capacity of 4 million books of books can be in one.
In theory, this understanding should mean the best and more advanced thinking. But do these windows translate the huge context into the value of business in the real world?
Since institutions weigh the costs of limiting infrastructure against potential gains in productivity and accuracy, the question remains: Do we open new limits in thinking about artificial intelligence, or simply extend the limits of symbolic memory without meaningful improvements? This article is examining in technical and economic implications, standard challenges and the development of institutional work that constitutes a large LLMS future.
Ascending the large context window models: noise or real value?
Why are the artificial intelligence companies racing to expand the lengths of the context?
Artificial intelligence leaders such as Openai, Google DeepMind and Minimax in the arms race to expand the length of context, which equals the amount of text that the artificial intelligence model can treat. The promise? Deeper understanding, fewer hallucinations and smoother interactions.
For institutions, this means that artificial intelligence can analyze the entire contracts, correct the large code rules, or summarize the long reports without breaking the context. Hope is that getting rid of solutions such as the generation of installation or the generation of recovery (RAG) can make Amnesty International’s work more smooth and more efficient.
Solve the problem of “needle-fish”
The problem of the needle in Histak indicates that it is difficult to identify the hidden information (the needle) within the Haystack groups. LLMS often misses the main details, which leads to inefficiency in:
- Search and Return of Knowledge: It fights artificial intelligence assistants to extract the most relevant facts from broad documentary warehouses.
- Legal and compliance: Lawyers need to track the dependencies of item through long contracts.
- Institutions analysis: Financial analysts risk the loss of decisive ideas buried in reports.
In the biggest context, Windows helps for models to keep more information and may reduce hallucinations. It helps improve accuracy and empowerment as well:
- Examination examination: One claim of 256K-Token can analyze the entire policy guide against new legislation.
- Medical literature creation: Researchers use 128K+ symbolic windows to compare the results of drug experience over decades of studies.
- Software Development: Correction of errors improves when artificial intelligence can scan millions of code without losing dependencies.
- Financial Research: Analysts can analyze full profit reports and market data in one inquiry.
- Customer Support: Chatbots offer with the tallest coach interactions of context.
Increasing the context window also helps the model reference better the relevant details and reduces the possibility of generating incorrect or fabricated information. The 2024 Stanford Study found that 128K Tukin models reduced hallucinations by 18 % compared to rag systems when analyzing integration agreements.
However, the first adoption of some challenges has been informed: JPMorgan Chase’s research shows how to perform the models badly in about 75 % of their context, with the collapse of performance in complex financial tasks to nearly 32 thousand symbols. Models are still widely struggling with long -term summons, often give priority to modern data on deeper visions.
This raises questions: Do the window of 4 million millions really reinforce thinking, or is it just an expensive expansion of memory? How much is this wide entry that the model already uses? Are the benefits outperforming the increasing mathematical costs?
Cost for performance: RAG against great claims: Which option wins?
Economic differentials to use the rag
RAG combines LLMS power with a retrieval system to bring in relevant information from an external database or document store. This allows the model to create responses based on the existing knowledge and the dynamic recovered data.
Since companies adopt artificial intelligence for complex tasks, they face a major decision: using huge claims with large context windows, or depend on Rag to bring dynamically relevant information.
- Large claims: Models with large symbolic windows address everything in one pass and reduce the need to maintain external retrieval systems and capture overlapping visions. However, this approach is calculated, with high inference costs and memory requirements.
- Rag: Instead of treating the entire document simultaneously, RAG only reclaims the most relevant parts before creating a response. This reduces the use of the distinctive symbol and costs, making it more applicable to real applications.
Comparison of the costs of reason
Although large claims simplify the workflow tasks, they require more GPU’s strength and memory, which makes them widely expensive. RAG -based methods often reduce, although demanding multiple retrieval steps, which leads to low inference costs without sacrificing accuracy.
For most institutions, the best method depends on the state of use:
- Do you need a deep analysis of documents? Large context models may work better.
- Do you need a cost -cost and effective Amnesty International for dynamic intelligence? The option is likely to be more intelligent.
It is a large window window of value when:
- The full text must be analyzed at once (for example: contract reviews, code review).
- The minimum of retrieval errors is very important (for example: organizational compliance).
- Cumin is less concerned about accuracy (for example: strategic research).
According to Google Research, the stock prediction models using Windows 128K surpassed 10 years of profit texts on RAT by 29 %. On the other hand, the internal test of the GitHub Copilot showed that completing the task 2.3x faster against the RAG Monorerobo Immigration.
Disproving the declining returns
Large context models: cumin, costs and ease of use
Although large context models provide great capabilities, there are limits to a really useful additional context. With the expansion of the context of Windows, three main factors play:
- Cumin: The more distinctive symbols, the slower inference. The windows of the greatest context can lead to a great delay, especially when it is needed in actual time.
- Costs: With each additional symbol processed, calculations rise. Increased infrastructure to deal with these large models can become expensive, especially for institutions with large size work burdens.
- The ability to use: with the growth of context, the model’s ability to “focus” effectively decrease the most relevant information. This can lead to ineffective treatment as less related data affects the performance of the model, which reduces returns for both accuracy and efficiency.
Google 4NAINING technology seeks to compensate for these differentials by storing compact representations of the context of the arbitrary length with limited memory. However, the pressure leads to the loss of information, and the models are combined to balance the immediate and historical information. This leads to a deterioration of performance and increased cost compared to the traditional piece.
The context window arms race needs the direction
While 4M token models are impressive, companies should use them as specialized tools instead of comprehensive solutions. The future lies in the hybrid systems that choose to be adaptive between rag and large demands.
Institutions should choose between large context and breaching models based on the complexity of thinking, cost and cumin. Large context windows are perfect for tasks that require deep understanding, while RAG is more expensive and effective for the most simple and realistic tasks. Institutions must set a clear cost limits, such as $ 0.50 per task, as large models can become expensive. In addition, large claims are more suitable for tasks that are not connected to the Internet, while RAG systems excel in actual time that require quick responses.
Emerging innovations such as Graphrag can increase the strengthening of these adaptive systems by integrating cognitive graphs with ways to retrieve traditional vectors that capture better complex relationships, improving accurate thinking and answering by up to 35 % compared to only methods. Modern applications by companies such as Lettria have shown dramatic improvements in resolution from 50 % with traditional RAT to more than 80 % using Graphrag within mixed retrieval systems.
Yuri Koratov also warns: “The expansion of context without improving thinking is similar to building the broader highway for cars that cannot be directed.The future of artificial intelligence lies in models that truly understand relationships across any context.
Rahul Raja is a LinkedIn employee software engineer.
Advitya Gemawat is a microsoft machine.
Don’t miss more hot News like this! Click here to discover the latest in Technology news!
2025-04-12 19:30:00