DeepSeek unveils new technique for smarter, scalable AI reward models
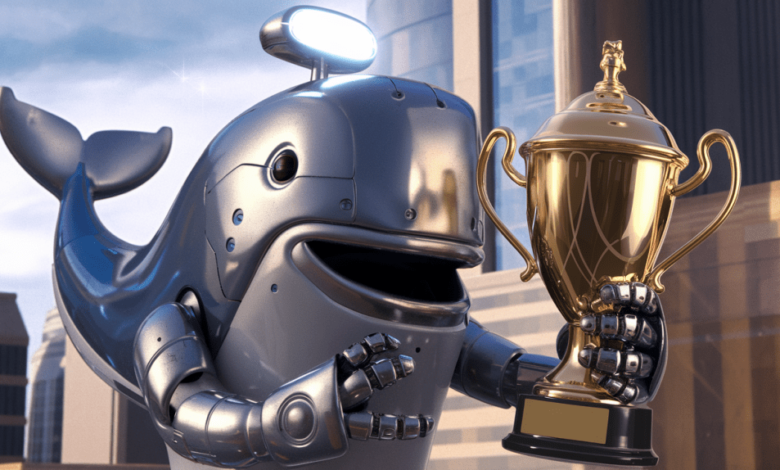
Join daily and weekly newsletters to obtain the latest updates and exclusive content to cover the leading artificial intelligence in the industry. Learn more
Deepseek Ai, a Chinese research laboratory, recognized his strong open source models such as Deepseek-R1, great progress in bonus modeling for LLMS models.
Their new style, self -controlling (SPCT), aims to create general and developed bonus models (RMS). This can lead to artificial intelligence applications for tasks and open fields where current models cannot capture the nuances and complications in their environment and users.
The decisive role and the current borders of the reward models
Learning for reinforcement (RL) has become a cornerstone in developing the latest LLMS. In RL, models are well adjusted based on counted feeding signals that indicate the quality of their responses.
The rewards models are the critical ingredient that provides these signs. Basically, RM works as a judge, evaluate LLM outputs and set a degree or “bonus” orientation of the RL process and knows LLM to produce more useful responses.
However, the current RMS often faces restrictions. They usually excel in narrow fields with clear rules or answers that can be easily verified. For example, modern thinking models such as Deepseek-R1 have underwent RL, where they were trained in mathematics and coding problems where the basic truth is clearly defined.
However, the creation of a bonus of complex, open or self -informative information in public fields still represents a major obstacle. In the paper that explains their new style, researchers at Deepseek Ai writes, “RM requires the generation of high -quality bonuses that go beyond the specific areas, where the bonuses standards are more diverse and complicated, and there is often no reference or explicit reality.”
They highlight four main challenges in creating a general RMS capable of dealing with the broader tasks:
- Input flexibility: RM must handle different input types and be able to assess one or more responses at one time.
- accuracy: Signs of accurate reward must be generated in various fields where the standards are complicated and the basic truth is not available.
- The ability to expand the time of reasoning: RM should produce high -quality bonuses when customizing more arithmetic resources during inferiority.
- Learning for developable behaviors: In order for the RMS scope to be effectively expanded at the time of reasoning, they need to learn behaviors that allow improving performance with the use of more account.
Rewards models can be widely classified through the “reward generation model” (for example, a single -degree numerical RMS output, RMS regulating text criticism) and “registration pattern” (for example, individual records are set for each response, and the couple chooses better than responses). These design options affect the suitability of the model for public tasks, especially Enterprise flexibility And the possibility Limbing the time of conclusion.
For example, the simple standard RMS struggles with the scaling time of inference because it will generate the same result frequently, while marital RMS cannot evaluate individual responses easily.
Researchers suggest that “Grum is” GRM), where the model creates text criticism and derives degrees from them, can provide flexibility and expansion required for public requirements.
Deepseek team conducted preliminary experiences on models such as GPT-4O and GEMMA-2-27B, and found that “some principles can direct the generation of bonuses within the appropriate criteria for GRMS, which improves the quality of rewards, which inspired us that the time of reasoning at the time of RM may be achieved by generating a generation of high ends and accurate criticisms.
RMS training to create their own principles
Based on these results, the researchers have developed self -monetary control (SPCT), which coaches GRM to create principles and criticisms based on queries and responsibilities.
Researchers suggest that the principles be “part of the generation of rewards instead of the pre -processing step.” In this way, GRMS can create principles while flying based on the task they evaluate and then create criticism based on principles.
“This transformation allows [the] The principles to be generated based on the inquiry and responses, the alignment is adaptive [the] The researchers write: “The process of generating bonuses, quality and principles of principles and corresponding criticism can be improved with postmatics training.”
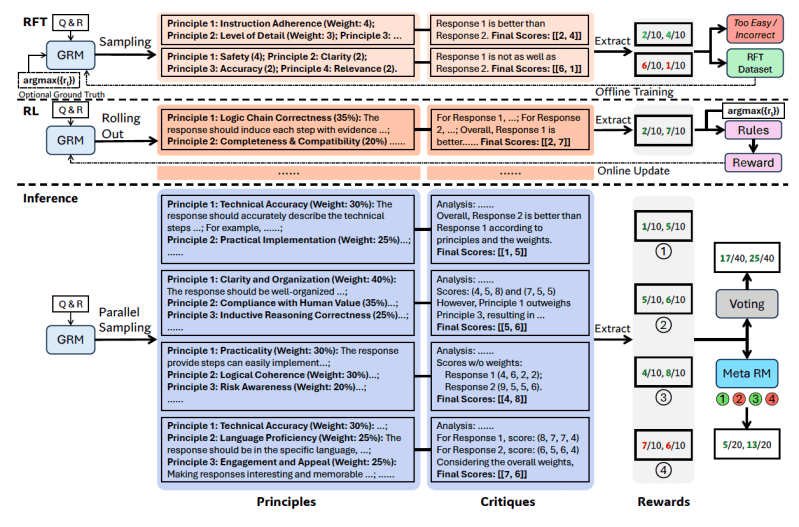
SPC includes two main phases:
- Soft rejection: This stage is trained to create principles and criticism of different input types using correct format. The model generates principles, criticisms and bonuses for the information/responses given. Paths (obstetric attempts) are not accepted unless the expected reward corresponds to the basic truth (determining the best response properly, for example) and rejecting them otherwise. This process is repeated and the model is adjusted on the elaborate examples to improve the capabilities of the principle/criticism.
- RL based on rules: At this stage, the model is more adjusted by the results -based learning. GRM generates principles and criticism for each query, and the reward signals are calculated based on simple accuracy rules (for example, did you choose the best known response?). Then the form is updated. This encourages GRM to learn how to generate effective principles and accurate criticism in a dynamic manner in a developed way.
“By taking advantage of RL online based on the bases, SPC GRMS allows learning the principles and criticisms that depend on adaptive in the way of input and responses, which leads to better results rewards in the public fields,” the researchers write.
To address the scaling challenge at the time of reasoning (obtaining better results with more account), researchers operate GRM several times for the same inputs, generating different groups of principles and criticism. The final reward is determined by voting (assembling sample scores). This allows the model to consider a broader set of perspectives, which leads to more accurate and accurate final provisions as it is provided with more resources.
However, some principles/criticisms that have been established are low quality or biased due to the restrictions of models or randomness. To address this, the researchers presented “Meta RM ” – Separate and lightweight RM trained specifically to predict whether the principle/criticism created by the initial GRM will lead to a correct final bonus.
During inference, Meta RM assesses samples created and liquidate low -quality provisions before the final vote, enhancing the scaling performance.
SPT mode in place with Deepseek-Grm
The researchers applied Spct to the GMMA-2-27B, Google OpenWight model, which creates Deepseek-GRM-27B. They evaluated it against many powerful main RMS (including LLM-AS-A-JugF, numerical RMS, and semi-lower RMS) and general models (such as GPT-4O and Nemotron-4-340b -Rward) via multiple criteria.
They found that Deepseek-GRM-27B surpassed the basic line trained methods of the same data. SPC greatly improved quality, decisively, the ability to expand at the time of reasoning compared to standard pressure.
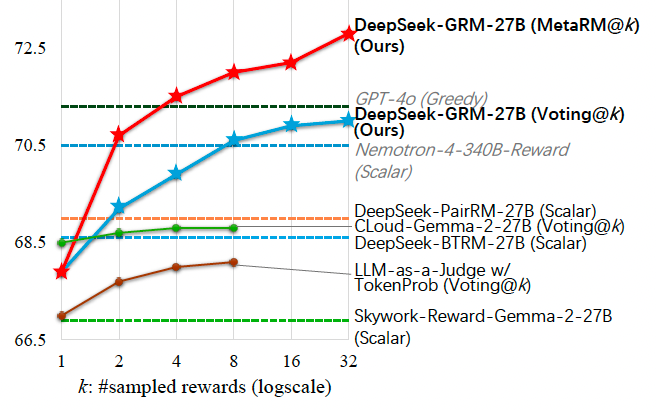
When limiting it at the time of inference by generating more samples, Deepseek-GRM-27B has increased significantly, bypassing a much larger models such as Nemotron-4-340b -Rward and GPT-4O. Meta RM has improved scaling and achieved the best results by filtering judgments.
“By sampling on a large scale, Deepseek-GRM can be more accurately governed by the principles of higher diversity, output bonuses with fine details,” researchers write.
Interestingly, SPC has shown a lower bias in various fields compared to numerical RMS, which was often well performed at check -in tasks but badly anywhere.
The effects of the institution
The development of more general and developed bonus models can be promising for the institution’s AI applications. Possible fields that can benefit from public RMS include creative tasks and applications as the model must adapt to dynamic environments such as advanced customer preferences.
Despite strong results, Deepseek-Grm still lags behind the numerical RMS numerical tasks that can be purely verified as the generation of frank thinking may be less efficient than direct registration. Efficiency is still a challenge compared to non -degenerative RMS.
Deepseek team suggests that future work will focus on deeper efficiency and integration improvements. As they conclude, “future trends can include combining GRMS into RL pipelines via the Internet as multi -use of bonus systems, exploring the joint time evaluation of politics, or as strong assessments in the non -connection models of basic models.”
Don’t miss more hot News like this! Click here to discover the latest in Technology news!
2025-04-08 22:33:00