Ensure Your Data is AI-Ready Today
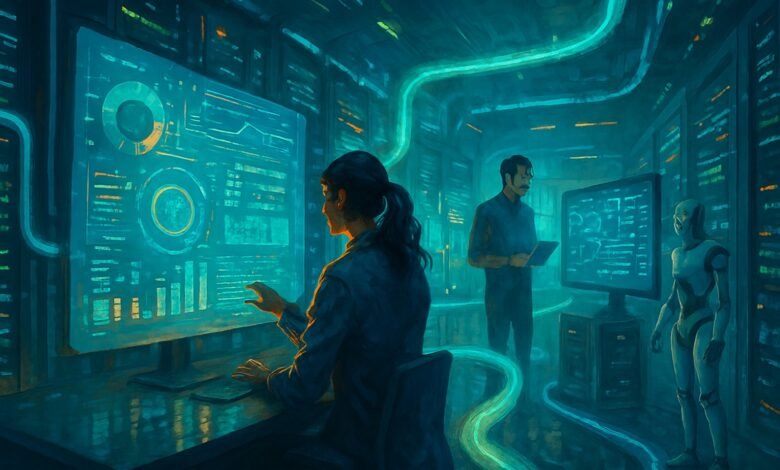
Make sure your data is ready today
Make sure your data is ready today By converting unorganized raw information into reliable, accurate and implemented assets. Artificial intelligence provides valuable only when trained in high -quality data. By picking up your audience’s attention, creating the desire for real transformation, stimulating immediate action, this article determines the basic steps to ensure that your data is really ready to spread artificial intelligence. Whether you are a business leader, a professional or a data world, make your data worthy of trust is not negotiable. Start building the most intelligent artificial intelligence tools by preparing your data in the right way.
Also read: Prepare your information technology infrastructure for artificial intelligence
Why clean and confidentially worthy data is a successful artificial intelligence basis
Artificial intelligence systems are only effective, such as the quality of the data they receive. Dirty or incomplete data leads to inaccurate predictions and automation failure. Unscreated or biased data training tools enhance errors instead of solving problems. This reduces confidence in both data and artificial intelligence system. The data preparation of Amnesty International exceeds the organization or simple storage. It takes a clear strategy for clean data sources, training, validation, and maintaining clean data sets.
Companies often store data via multiple silos – ERP systems, CRMS, data tables, and cloud applications – in formats and their own standards. Without appropriate integration, it becomes duplication and turns major issues. When data sources are not aligned or cleaned regularly, they make misleading decisions and wrong artificial intelligence results.
Also read: Organizational sciences meet artificial intelligence
The biggest challenges in making the data ready
Organizations face several obstacles when preparing their data for artificial intelligence applications:
- Lack of consistency: Data collected from different structures, label agreements or measurement units often vary.
- Inclusion: Lost values or old records can distort models and reduce accuracy.
- prejudice: Historical data can reflect social, cultural or operational biases, which are encrypted in machine learning models.
- Security risks: The wrong or classified information can increase the concerns of data privacy or compliance violations.
These issues not only waste resources, but also delay the project schedules. The artificial intelligence solution trained on defective inputs cannot be trusted to provide accurate or fair outputs, making data enhancement a first vital step.
Steps to make sure
Data preparation is an organized process that focuses on improving quality, tracking, expansion and privacy. Here are the critical steps that must be followed:
1. Conducting a comprehensive data audit
Start by selecting all data sources inside and outside the institution. Each source of accuracy, timing and importance. During this process, a sign of non -organized, semi -organized, and organized data accordingly. This stage helps you imagine a full data scene and build a road map for improvements.
2. Standardization of data formats and their definitions
Unification creates harmony through data entries. The choice of a unified scheme for data fields such as names, addresses, currencies, product identifiers, or temporal nature reduces confusion. Institutions must also develop a business report – a central reference for consistent definitions across the teams. When everyone talks about the same data language, artificial intelligence training becomes more reliable.
3. Cleansing and verifying continuously
Cleaning includes determining repetitions, removing incorrect values, filling lost fields whenever possible. Implement the algorithms or manual reviews based on the type of data. Checking health verification such as consistency rules, verification of coordination, and relationships of relationship to improve confidence at each level. These rules should be constantly imposed using data quality pipelines and machine checks.
4. Crack data silos
Data silos limit knowledge of knowledge and learn about knowledge within isolated business units. Incorporation of different systems through application programming facades, data lakes or cloud platforms to unify access. Cooperation through approval and giving the main stakeholders encouraged the necessary vision to contribute a useful contribution. Unified data allows artificial intelligence models to draw visions of a broader and varied source gathering.
5. Monitoring bias and enhancing fairness
Bias in data groups can lead to unfair or unethical decisions. Science and data analysis to ensure that it reflects a wide and comprehensive set of values and demography. Various representation improves the model’s ability to deal with real world scenarios across different user groups. Use regular audits to detect unintended connections that can cause bias or discrimination.
6. Properly secure the data and control
The restoration of artificial intelligence not only related to the structure, but also includes policies. Prepare data governance systems that define data, which can be accessed, and how it can be used. Carry out a strong encryption, roles -based access, and audit paths. Track the data rates so that each transformation and enrichment activity can be tracked to its source. These practices provide data and ensure compliance with international frameworks such as GDP or CCPA.
7. Use signs and explanation tools for data for accuracy
Training artificial intelligence models on data sets called the form of the model and the vival of tracking. Use illustrating comments platforms to mark photos, videos, text, or sound accurately. For example, in computer vision applications, the surrounding squares or retail tools help to determine the object’s boundaries so that the model learns to know the objects better. In the treatment of the natural language, signs of parts of speech or feelings ensure a better linguistic context. The data that is well named reduces noise and enhances the efficiency of the model.
Also read: How will artificial intelligence (AI) change internal audits?
Descriptive data provides a context that enhances the value of the data. It describes where the data came from, how it was changed, and how it should be used. Adding descriptive data allows better discovery, management and analysis. Signs such as the source, date, owner, or type of content simplify indexing and research operations. Artificial intelligence models usually trained with data -rich data collections better lead to the context with the context with the content. Metadata also supports transparency, review, and building confidence in artificial intelligence outputs.
Technology can accelerate the success of the data. Think of using the following categories of tools:
- ETL/elt: Data pipeline tools such as Talend, Informatica or Apache Airflow Extract Data, and turn them into useable formats, and download them into modeling warehouses.
- Data quality platforms: Pits such as Ataccama or Talend provides data, monitoring and enrichment to constantly improve quality.
- ML data description tools: Pits such as Supernotate, Labelbox or Scale AI help comment on large amounts of data efficiently.
- Data lakes: Uniform like Azure Data Lake, Aws Lake Formation or Snowflake focus on information from multiple sources and enables easy easy treatment.
These platforms produce immediate results when implementing them properly and integrate with broader data governance policies.
Make the success of artificial intelligence is inevitable with the first data approach
Preparing for artificial intelligence begins before choosing artificial intelligence models or algorithms. The performance of these models and fully confidence depends on the quality and stability of input data. By conducting audit operations, cleaning practices, governance, and marking, the teams can open the full force of their data assets. Broken or outdated data systems can no longer stand the competitiveness. The institutions that are now investing in the structure of data worthy will reap the benefits of active and smart automation in less time and better results.
Also read: Understanding the risks of artificial intelligence safety before investing
Conclusion: Building confidence in your data and let artificial intelligence work for you
Trust is the basis for every successful Amnesty International initiative. This confidence begins with responsible, clean, organized and unbiased data. When your systems are concerned with the data strategy from one to the party-from the moment when the data is collected to the point that is fed in a model-every decision derived from artificial intelligence becomes more accurate, timely and effectively. Your data does not need to be enormous, but it should be meaningful. The future of the success of artificial intelligence is not only related to a country or models – it is related to data confidence.
Reference
Parker, Professor Philip M. , PhD Global view 2025-2030 for artificial intelligence in health care. Insead, March 3, 2024.
Khang, Alex, Editor. Innovations driven by artificial intelligence in digital health care: emerging trends, challenges and applications. Igi Global, February 9, 2024.
Singla, Babita, et al. , Editors. A revolution in the health care sector with artificial intelligence. Igi Global, July 26, 2024.
Topol, Eric J. Deep Medicine: How can artificial intelligence make human health care again. Basic books, 2019.
Nelson, John W., Editor, and others. Using predictive analyzes to improve health care results. 1st ED. , Apress, 2021.
Subbhuraam, Vinithasree. Predictive analyzes of health care, Volume 1: Transfer the Future of Medicine. First edition, Institute for Public Publishing, 2021.
Kumar, Abhishek, and others, editors. Development of predictive analyzes in health care: The new Amnesty International Technologies for Actual Time. Engineering and Technology Corporation, 2022.
Tetteh, Hassan A. More intelligent health care with artificial intelligence: harnessing military medicine to revolutionize health care for all, everywhere. Forbesbooks, November 12, 2024.
Lori, Tom. Artificial Intelligence in Health: The Leader Guide to Winning in the era of new smart systems. First edition, HIMSS, February 13, 2020.
Holly, Kerry, and Manish Matore. LLMS and AI Tawylidi Healthcare: The following limits. First edition, O’Railly Media, September 24, 2024.
Holly, Kerry, and Siopo Baker MD Amnesty International Health Care: Amnesty International Applications in Business and Clinical Management for Health. First edition, O’Railly Media, May 25, 2021.
Don’t miss more hot News like this! Click here to discover the latest in AI news!
2025-04-11 13:10:00