From motor control to embodied intelligence
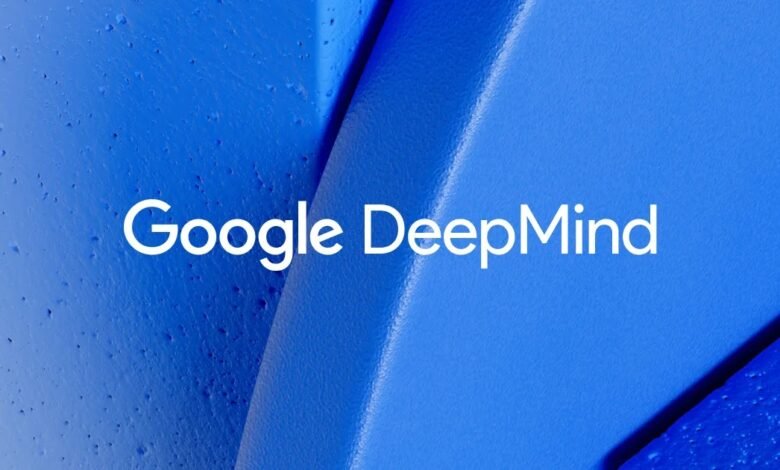
research
Using human and animal suggestions to teach robots to heat a ball, simulate human characters to carry boxes and play football
Learning human characters to pass the path of an obstacle through experience and error, which can lead to special solutions. Hess, and others. “The emergence of movement behaviors in rich environments” (2017).
Five years ago, we faced the challenge of teaching a fully articulated human personality to pass obstacles. This showed what the reinforcement learning (RL) can achieve through experience and error, but it also highlighted two challenges in the solution Incarnate intelligence:
- Reuse the previously learned behaviors: A large amount of data was needed for the “descent from the ground”. Without any preliminary knowledge of the force that must be applied to each of its joints, the worker began with random translation in the body and quickly falls on the ground. This problem can be reduced by reusing the previously learned behaviors.
- Distinctive behaviors: When the customer finally learned to move in obstacles, do this with abnormal (albeit) movement patterns that will be impractical for applications such as robots.
Here, we described a solution to both challenges called NPP alternatives (NPMP), which involves learning directed with the patterns of movement derived from humans and animals, and we discuss how this approach is used in our human football paper, published today in science robots.
We also discuss how this same approach allows the complete manipulation of the human body of vision, such as humans that carry an object, and automatic control in the real world, such as the robot that plays a ball.
Data distance in controlled engine alternatives with NPMP
NPMP is a general -purpose engine control unit that translates short horizon intentions into low -level control signals, training them in non -communication mode or via RL by imitating movement data (MCAP), registered with human beings or animals that lead to importance.
An agent learns to imitate the MCAP path (as shown in gray).
The model contains two parts:
- The encrypted that takes a future path and presses it in the intention of a engine.
- A low -level control unit produces the following procedure given the current position of the agent and this kinetic intention.
Our NPMP model first represents reference data in a low -level control unit (left). This low -level control unit can then be used as a connection and operation engine control unit on a new (right) mission.
After training, a low -level control unit can be reused to learn new tasks, as a high -level control unit is improved to direct the engine intentions directly. This effective exploration allows – since coherent behaviors are produced, even with the intentions of the engine from which samples were randomly taken – and restricting the final solution.
Coordination of the emerging team in human football
Football was a long challenge for embodied intelligence research, which requires individual skills and playing the coordinated team. In our latest work, we used NPMP as before learning movement skills.
The result was a team of players who had learned to chase the ball, to finally learn coordination. Previously, in a study with simple embodiment, we have shown that coordinated behavior can appear in teams competing with each other. NPMP allowed us to monitor a similar effect but in a scenario that requires more advanced engine control.
The agents first simulate the movement of football players to learn NPMP (higher). Using NPMP, agents learn soccer skills (bottom).
Our agents have skills including the graceful movement, traffic and the division of labor as shown in a set of statistics, including standards used in sports analyzes in the real world. Players show both high -frequency graceful monitoring and long -term decisions that involve expecting the behavior of his teammates, which leads to coordination of team play.
An agent learns to play football competitively using the RL Multi-Agent.
Tampering with a full body and cognitive tasks using vision
Learning to interact with things using weapons is another difficult challenge. NPMP can also enable this type of full body manipulation. With a small amount of MoCAP data to interact with the boxes, we can train an agent to carry a box from one site to another, using only a singer vision with a few bonus signal:
With a small amount of MOCAP data (higher), our NPMP approach can solve a task (bottom).
Likewise, we can teach the agent to pick up the balls and throw them:
Simulation of human hunting and throwing the ball.
Using NPMP, we can also treat maze tasks that include movement, perception and memory:
Human simulation collection of blue fields in a maze.
Safe and effective control of robots in the real world
NPMP can also help control real robots. The presence of well -organized behavior is very important for activities such as walking over raw terrain or dealing with fragile things. Robot tense suggestions can be damaged by the same robot or its surroundings, or at least to drain the battery. Therefore, a great effort is often invested in designing learning goals that make the robot do what we want while behaving in a safe and effective way.
As an alternative, we have investigated whether the use of the briring derived from biological movement can give us regular, natural and reusable movement skills for robots with legs, such as walking, running and operating suitable for publication on robots in the real world.
Starting with the MoCAP data from humans and dogs, we adapted the NPMP approach to train skills and simulation control units that can then be published on real human robots (OP3) and a quarter -quadruple (iP.) successive. This allowed the guidance of robots by a user via the control stick or the diameter of the ball with a targeted and powerful site.
The movement skills of Anymal robot are learned by imitating the MCAP dogs.
Movement skills can then be reinforced to control walking and ball.
Benefits of using nervous probability engine allowances
In short, we used the NPMP skill model to learn complex tasks with human characters in simulation and robots in the real world. NPMP packages are low -level motion skills in a reusable way, facilitating learning useful behaviors that are difficult to discover through experience and unorganized error. Using motion capture as a source of previous information, it bends the motor control learning towards natural movements.
NPMP allows embodied factors to get to know more quickly using RL; To learn more natural behaviors; To know safer, effective and stable behaviors suitable for robots in the real world; And to collect control of the entire body with the longest horizontal cognitive skills, such as teamwork and coordination.
Learn more about our work:
Don’t miss more hot News like this! Click here to discover the latest in AI news!
2022-08-31 00:00:00