Handling Expert Shift and Intermittent Feedback
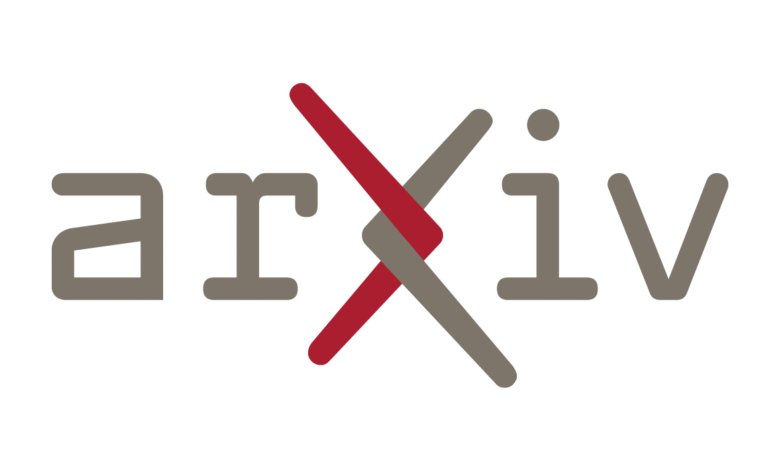
View the PDF file from the paper entitled Interactive Interactive Learning: Treating Experts and Intermittent Comments, by Michelle Chao and 4 other authors
PDF HTML (experimental) view
a summary:In interactive traditional learning (IL), the estimate of uncertainty provides an IE ROBOT to counter the distribution attacks that were faced during the publication by searching for additional notes from an expert (i.e. human) via the Internet. The mechanisms of using previous businesses such as division or the leaked Monte Carlo to measure when IL policies with a black box are uncertain; However, these methods can lead to excessive confidence estimates when facing publishing time distribution attacks. Instead, we claim that we need algorithms to measure uncertainty that can benefit from the expert comments that were received during the publication time to adapt uncertainty in the online robot. To address this, we are based on online corresponding prediction, which is a distribution -free way to create online prediction periods given a stream of terrestrial truth stickers. Human labels, however, intermittently in interactive IL preparation. Thus, on the side of the matching prediction, we offer the new ingredient measuring algorithm called the IQT tracker (IQT) that benefits from a possibility of intermittent signs, maintains non -submitted coverage guarantees, and experimentally achieves the required coverage levels. On the interactive IL side, we develop Contiversalgagger, a new approach where the robot uses prediction periods that have been calibrated by IQT as a reliable scale for uncertainty at the time of publication to inquire actively to get more expert reactions. We compare compatibility with the pre -dagger methods in the uncertainty in the scenarios where the distribution transformation is present (not) due to the changes in the expert’s policy. We find that in the publishing operations simulating and devices on 7DOF robots, Continmalgger discovers high uncertainty when experts turn and increase the number of interventions compared to basic lines, allowing the robot to quickly learn new behavior.
The application date
From: Michelle Chao [view email]
[v1]
Fri, 11 Oct 2024 14:27:56 UTC (120,993 KB)
[v2]
Tuesday, April 29, 2025 12:17:52 UTC (130,507 KB)
Don’t miss more hot News like this! AI/" target="_blank" rel="noopener">Click here to discover the latest in AI news!
2025-05-01 04:00:00