Kirill Solodskih, Co-Founder and CEO of TheStage AI – Interview Series
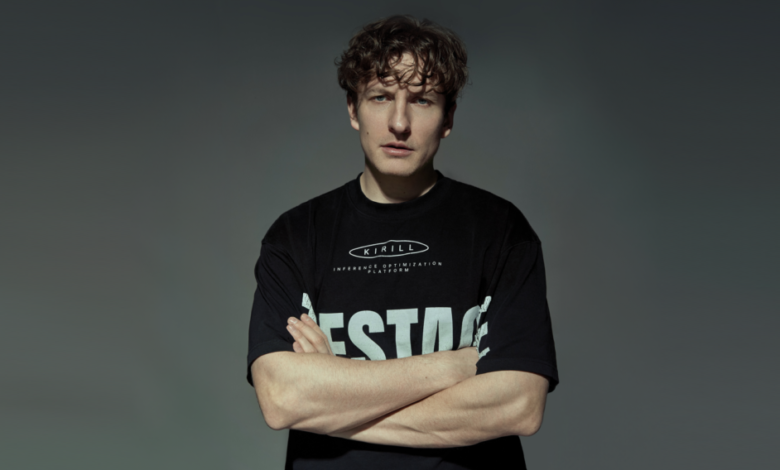
Kirill Solodskih, PhD, is the co -founder and CEO of Thestage AI, as well as an artificial intelligence researcher and a businessman with more than a decade of experience in improving nervous networks for business applications in the real world. In 2024, he participated in the founding of Thestage AI, which received $ 4.5 million of financing to fully nerve network speeding through any devices platform.
Previously, as a team leader in Huawei, Kirill led the acceleration of the Qualcomm NPUS, which contributed to the performance of the P50 and P60 smartphones and gained multiple patents for its innovations. His research was shown in leading conferences such as CVPR and ECCV, where he received prizes and recognition at the level of industry. Podcast also hosts improving artificial intelligence and inference.
What inspired you to participate in the founding of Thestage AI, and how did you move from academic circles and research to address improvement in reasoning as an founder of starting start?
The foundations of what became the same in my work in Huawei, where I was deep in automating publishing and improving nerve networks. These initiatives have become the basis for some of our leading innovations, and this is where you saw the real challenge. Training the model is something, but getting it works efficiently in the real world and making it accessible to users is something else. Publishing is the bottle neck that hinders many great ideas from reaching life. To make something easy to use like ChatGPT, there are many rear challenges that involve them. From a technical perspective, improving the nerve network revolves around reducing parameters while maintaining high performance. It is a problem with difficult mathematics with a large space for innovation.
Improving manual inference has always been the bottleneck in artificial intelligence. Can you explain how Thestage Ai automates this process and why is the game change?
Thestage AI takes the main bottle neck in artificial intelligence: manual pressure and accelerate nerve networks. Neurological networks have billions of parameters, and know those that must be removed to improve the impossible performance almost by hand. Anna (automatic neurological network analyst) This process is automated, and the layers that cannot be excluded from improvement are determined, similar to how the postal code compresses for the first time.
This changes the game by making artificial intelligence adoption faster and more affordable. Instead of relying on expensive manual processes, startups can automatically improve models. This technology gives companies a clear vision of performance and cost, ensuring efficiency and expansion without guessing.
Thestage AI claims to reduce the costs of inference by up to 5X – What makes your improvement technology very effective compared to traditional methods?
Thestage AI reduces the costs of up to 5X with an improvement approach that exceeds traditional methods. Instead of applying the same algorithm on the entire nerve network, Anna divides it into smaller layers and decides the algorithm that will apply for each part to submit the required pressure with an increase in the quality of the form. By combining smart sporting reasoning with effective approximately, our approach is very developmental and makes adopting artificial intelligence easier for companies of all sizes. We also merge the flexible code to improve networks for specific devices such as iPhone or NVIDIA GPU. This gives us more performance control well, increasing speed without losing quality.
How is the acceleration of inferences from artificial intelligence compares to the original translator of Biturche, and what are the advantages that artificial intelligence developers offer?
Thestage AI is to direct it beyond the original Pytorch translator. Pytorch uses a “timely” assembly method, which combines the model every time it is turned on. This leads to long start times, sometimes it takes minutes or even longer. In developable environments, this can create shortcomings, especially when new online graphics processing units need to deal with an increase in the user download, causing a delay that affects the user experience.
On the contrary, Thestage AI allows pre -materials in advance, so as soon as the model is ready, it can be published immediately. This leads to faster alert, improving service efficiency, and providing costs. The developers can spread and expand the scope of artificial intelligence models faster, without traditional assembly bottlenecks, which makes them more efficient and responsive to highly requested use cases.
Can you share more about the QLIP set for Thestage AI and how to enhance the performance of the model while maintaining quality?
QLIP, TheStage AI, is the Python Library that provides an essential group of primitive to build new improved algorithms at a speed designed for different devices, such as graphics processing units and NPUS. The set of tools includes components such as quantitative measurement, pruning, specifications, assembly, and service, all of which are important to develop effective and developed artificial intelligence systems.
What distinguishes Qlip is its flexibility. Artificial intelligence engineers allow the initial model and the implementation of new algorithms with a few lines of code. For example, the modern Amnesty International Conference paper on measuring nerve networks can be converted into a work algorithm using QLIP beginnings in minutes. This makes it easy for developers to integrate the latest research in their models without being kept through solid frameworks.
Unlike the traditional open -source traditional frameworks that restrict you to a fixed set of algorithms, QLIP allows anyone to add new improvement techniques. This ability to adapt the difference to stay at the top of the sophisticated scene quickly, which improves performance while ensuring flexibility for future innovations.
It has contributed to the work frameworks of the quantity of artificial intelligence used in the Huawei P50 and P60 cameras. How did this experience formed your approach to improving artificial intelligence?
My experience in working on the work frameworks of Amnesty International for Huawei’s P50 and P60 valuable visions on how to simplify the improvement and expand its scope. When I first started with Pytorch, working with the graph for the full implementation of the nerve networks was rigid, and the manually quantitative algorithms had to be performed, layer after another. In Huawei, I built an automatic operation framework. You can simply enter the form, and will automatically create a quantitative measurement code, and eliminate manual work.
This led me to realize that automation in improving artificial intelligence revolves around enabling speed without sacrificing quality. She became one of the algorithms she developed and got a patent necessary for Hoyoui, especially when she had to move from Kirin processors to Qualcomm due to the sanctions. The team was allowed to quickly adapt nerve networks with Qualcomm brown without losing performance or accuracy.
By simplifying the process and automating the operation, we reduce the development time from more than a year to only a few months. This had a significant impact on the product used by millions and formed an approach to improvement, focusing on speed, efficiency and minimum quality loss. This is the mentality that I bring to Anna today.
Your search is shown in CVPR and ECCV – What are some of the main breakthroughs in the efficiency of artificial intelligence that you are proud of more?
When I was asked about my accomplishments in the efficiency of artificial intelligence, I always think about the paper that was chosen for an oral offer in CVPR 2023. The choice of an oral offer in such a conference is rare, as only 12 sheets are chosen. This adds to the fact that the immunic artificial intelligence usually dominates the lights, and our paper has taken a different approach, focusing on the sports side, specifically the analysis and pressure of nerve networks.
We have developed a method that helped us understand the number of parameters that the nerve network really needs to work efficiently. By applying technologies from functional analysis and moving from separate to continuous formulation, we were able to achieve good pressure results while maintaining the ability to combine these changes again into the model. The paper also introduced many new algorithms that the community did not use and found more application.
This was one of my first papers in the field of artificial intelligence, and most importantly, it was the result of our team’s team effort, including the founders of my participation. It was a great milestone for all of us.
Can you explain how integrated nervous networks (INNS) work and why is an important innovation in deep learning?
Traditional nerve networks use fixed matrices, similar to Excel schedules, where the size and parameters are determined in advance. However, INNS describes networks as continuous functions, providing much more flexibility. Think of it like a blanket with pins at different altitudes, and this represents the ongoing wave.
What makes Inns exciting is its ability to “pressure” or “expand” dynamically based on the available resources, similar to how the analog signal is numbered into a sound. You can reduce the network without sacrificing quality, and when needed, expand it again without re -training.
We have tested this, and while traditional pressure methods lead to great quality, INNS maintains quality close to the original even with extreme pressure. Mathematics behind it is unconventional to the artificial intelligence community, but the real value lies in its ability to provide strong and practical practical results with the minimum effort.
Thestage AI has worked on quantum steel algorithms – how do you see quantum computing playing a role in improving artificial intelligence in the near future?
When it comes to quantum computing and its role in improving artificial intelligence, the main meals are that quantum systems provide a completely different approach to solving problems such as improvement. Although we have not invented quantum steel algorithms from zero point, companies like D-WAVE provide Python libraries to build quantum algorithms specifically for separate improvement tasks, which are ideal for quantum computers.
The idea here is that we do not download a nervous network directly to the quantum computer. This is not possible with current architecture. Instead, we are closer to how nerve networks act under different types of deterioration, which makes them fit with a system that the quantum chip can do.
In the future, quantum systems can expand the scope of networks and improve them accurately fight traditional systems for matching. The quantum systems are in the integrated parallel, which can only mimic classic systems using additional resources. This means that quantum computing can greatly accelerate the improvement process, especially as we learn how to design larger and more complex networks effectively.
The real capabilities in the use of quantum computing come to solve huge and complex improvement tasks and convert teachers into smaller and more management groups. With technologies such as quantum and visual computing, there are extensive possibilities to improve artificial intelligence that much exceeds what traditional computing can provide.
What is your long -term vision of Thestage AI? Where do you think that improving reasoning is heading in the next 5 to 10 years?
In the long run, Thestage AI aims to become a global center for the model where anyone can easily access an improved nerve network with the required features, both for a smartphone or any other device. The goal is to provide a drag and escape experience, as users enter their parameters and the system automatically creates the network. If the network is not already present, it will be created automatically using Anna.
Our goal is to make nervous networks directly on user devices, and reduce costs by 20 to 30 times. In the future, this may completely cancel the costs, as the user device will deal with the account rather than relying on cloud servers. This, in addition to developments in the compression of the model and the acceleration of devices, can make the spread of artificial intelligence more efficient.
We also plan to integrate our technology with hardware solutions, such as sensors, chips and robots, for applications in areas such as independent driving and robots. For example, we aim to build artificial intelligence cameras capable of working in any environment, whether in space or under harsh conditions such as darkness or dust. This would make Amnesty International to be used in a wide range of applications and allow us to create solutions for specific devices and use.
Thank you for the wonderful interview, readers who want to know more, visit Thestage AI.
Don’t miss more hot News like this! Click here to discover the latest in AI news!
2025-04-15 16:31:00