Meet Open-Qwen2VL: A Fully Open and Compute-Efficient Multimodal Large Language Model
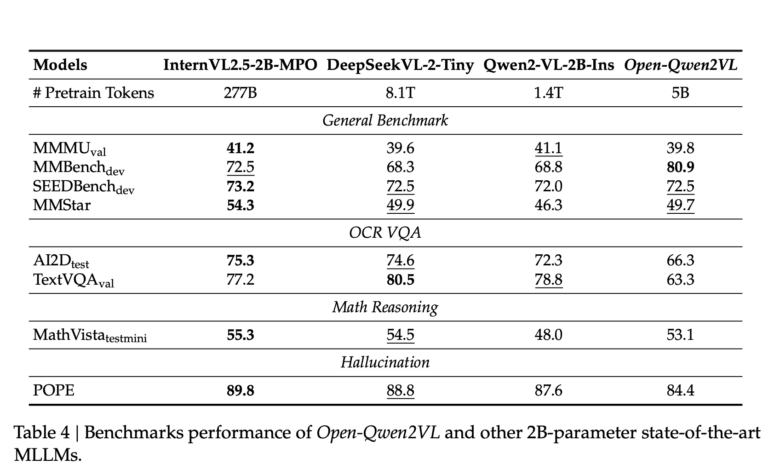
MLLMS models have developed the integration of visual and text methods, allowing progress in tasks such as illustrations of photos, answering visual questions, and interpretation of documents. However, the symmetrical copies and the development of these models are often hindered by a lack of transparency. Many MLLMS are not released to the latest MENS model, including training code, data stimulating methodologies, and pre -data groups. Moreover, the large computer resources required to train these models constitute a large barrier, especially for academic researchers with limited infrastructure. This lack of access impedes its cloning and slows down the deployment of new technologies within the research community.
Researchers from the University of California in Santa Barbara, Beettenanis and Nvidia Open-SWEN2VL, a multimedia language model consisting of 2 billion, which was previously trained on 29 million pairs of pictures using about 220 A100-40g GPU watches. It was cooperatively developed by researchers from the University of California in Santa Barbara, Beettenance, and NVIDIA Research, QWEN2VL Open to treat cloning and resource restrictions in MLLM research. The project provides a full range of open source resources, including the training code base, data filtering texts, pre -training data for WEBDATASET format, and base inspection points and instructions. This comprehensive version aims to support the transparent experience and develop the method in the field of multimedia learning.
Open-SWEN2VL depends on the backbone QWEN2.5-1.5B-Instruct LLM, along with the Siglip-SO-400M vision encrypted. The average average visual display of roaming reduces the number of visible symbols from 729 to 144 during training, which improves mathematical efficiency. The number of the distinctive symbol is increased to 729 during the control stage (SFT). Low to high resolution strategy maintains images with improving resource use.
To increase the enhancement of training efficiency, QWEN2VL carries out a multi -media sequence, allowing the sequence of multiple pictures text to a sequence of about 4096 symbols, thus reducing filling and account. Vision encryption parameters remain frozen during training to maintain resources and are optionally heterogeneous during SFT to improve the power performance.
QWEN2VL is trained on only 0.36 % of the number of the distinctive code used in QWEN2-VL, but it shows the same or superior performance across several criteria. The model achieves 80.9 degrees on MMBENCH, and performs competitive on SuPBench (72.5), MMSTAR (49.7), and Mathvista (53.1). Huritage studies indicate that merging a small sub -group (5 meters samples) of high -quality pairs that are nominated using MLM techniques can lead to measurable performance improvements, highlighting the importance of data quality at the audio level.
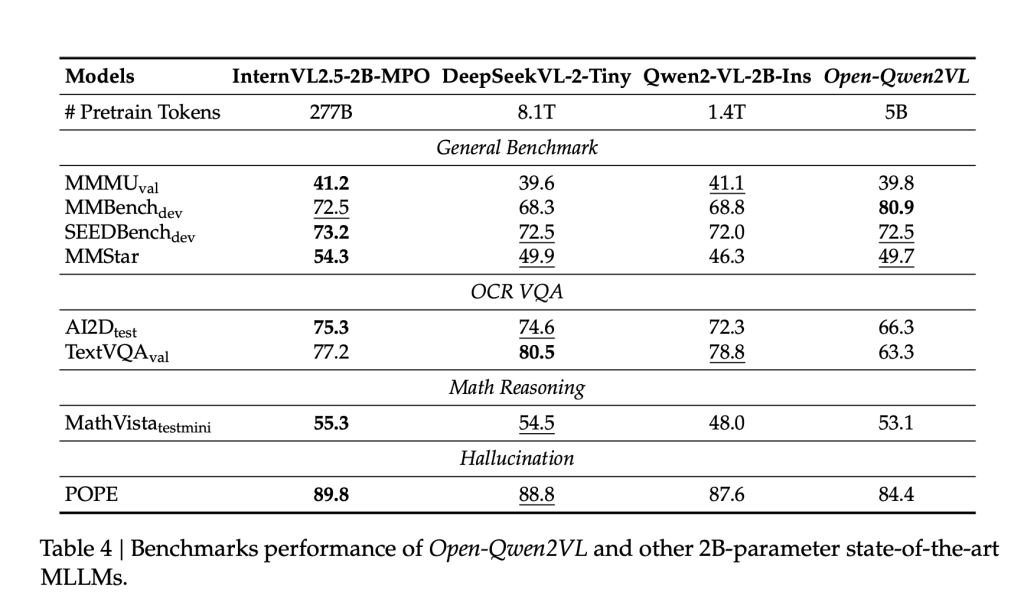
In addition, Open-SWEN2VL displays the few multimedia learning capabilities in the context. When evaluating data collections such as GQA and TextvQA, the model shows accuracy gains from 3 % to 12 % of 0 -shot scenarios to 8 shots. Micro-performance scales can be predicted with the size of the instruction control data set, with performance gains that reach about 8 meters from the Mammoth-VL-10M data set.
QWEN2VL Open-SWEN2VL offers a repetitive and effective pipeline to train large multimedia language models. By processing the restrictions of the previous models systematically in terms of openness and calculating the requirements, it allows the broadest participation in MLM research. Form design options – including effective visual symbol processing, multi -media -sequence packaging, and choosing wise data – choose a front -to -anchor pathway for academic institutions aimed at contributing in this field. QWEN2VL Open-SWEN2VL creates a cloning basis line and provides a basis for future work on the developed and high-performance MLLMS within restricted accounting environments.
Payment Paper, model, data and symbol. All the credit for this research goes to researchers in this project. Also, do not hesitate to follow us twitter And do not forget to join 85k+ ml subreddit.
🔥 [Register Now] The virtual Minicon Conference on Open Source AI: Free Registration + attendance Certificate + 3 hours short (April 12, 9 am- 12 pm Pacific time) [Sponsored]
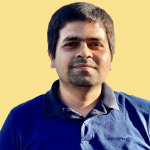
Asif Razzaq is the CEO of Marktechpost Media Inc .. As a pioneer and vision engineer, ASIF is committed to harnessing the potential of artificial intelligence for social goodness. His last endeavor is to launch the artificial intelligence platform, Marktechpost, which highlights its in -depth coverage of machine learning and deep learning news, which is technically sound and can be easily understood by a wide audience. The platform is proud of more than 2 million monthly views, which shows its popularity among the masses.

2025-04-04 05:15:00