Meta FAIR advances human-like AI with five major releases
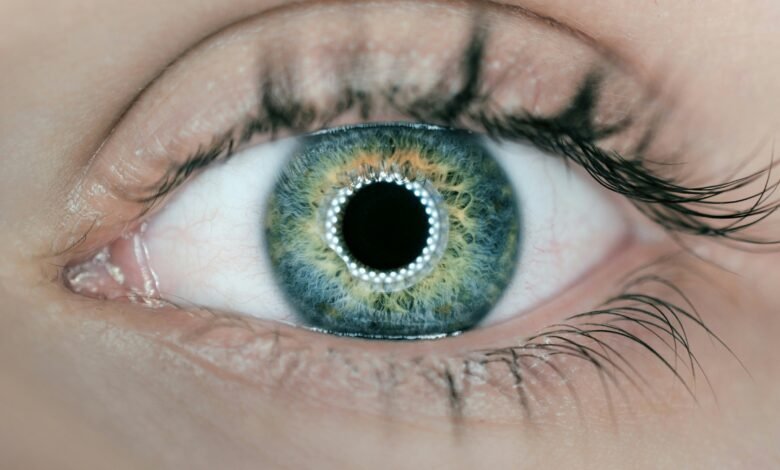
The Fair Intelligence Research team in Meta has announced five projects that offer the company’s endeavor to advanced machine intelligence (AMI).
The latest versions of Meta focus greatly on enhancing artificial intelligence – the ability of machines to process and interpret sensory information – as well as developments in language modeling, robotics, and collaborative intelligence agents.
Meta stated that its goal involves creating machines “capable of obtaining, processing and interpreting sensory information around the world around us, and they can use this information to make decisions with intelligence and speed -like speed.”
The five new publications represent the various but interconnected efforts towards achieving this ambitious goal.
Cognition encryption: Meta sharpens the “vision” of artificial intelligence
One of the basic things for new versions is the cognition encryption, described as a wide -ranging vision designed to excel across various photo and video tasks.
The vision encoders act as “eyes” for artificial intelligence systems, allowing them to understand visual data.
Meta highlights the increasing challenge of building encoders that meet the requirements of advanced artificial intelligence, which require capabilities that address vision and language, dealing with both images and videos effectively, and remain strong under difficult conditions, including potential hostilities.
The perfect encrypted, according to Meta, should recognize a wide range of concepts with a distinction between fine details – which puts examples such as the discovery of “STINGY LUROROWED” at the bottom of the sea, with a small golfish in the background of a picture, or hunting the roaming Agouti on a wildlife camera in night vision. “
Meta claims that the perception of the perception achieves “an exceptional performance on the classification of images and video zero and retreat, bypassing all the models in open and owned sources of such tasks.”
Moreover, its cognitive strengths are said to be well translated into language tasks.
When compatible with a large language model (LLM), it is said that the encrypted surpasses other vision symbols in areas such as answering visual questions (VQA), clarification designation, understanding of documents, and grounding (linking the text to specific image areas). According to what was stated, performance is enhanced by traditional traditional tasks for LLMS, such as understanding spatial relationships (for example, “if there is an object behind another”) or the movement of the camera for an object.
Meta said: “With the start of perception of new applications, we are excited to see how advanced vision capabilities will enable more capable artificial intelligence systems,” Meta said.
Penm Language Model: Open Research in Vision
The completion of the encrypted is a perception of perception (PLM), which is an open and linguistic cloning model that aims to complex visual identification tasks.
PLM has been trained using extensive artificial data with open language data groups, explicitly without distortion of knowledge from external ownership models.
By identifying the gaps in the current video understanding data, the fair team collected 2.5 million new samples with a human sign that focuses on answering accurate video questions and spatial and temporal comment. Meta claims that this is the “largest data collection of its kind so far.”
PLM is presented in parameters ’versions 1, 3 and 8 billion, meeting the needs of academic research that require transparency.
Besides the models, Meta releases Plm-Videobench, a new standard specifically designed to test capacity often lacks current standards, that is, “understanding precise activity and spatial logic on Earth.”
Meta hopes to enable a mixture of open models, a large data collection, and the difficult standard of open source.
Meta 3D location: giving robots of circumstantial awareness
Blocking the gap between language orders and physical action is the location of the Meta 3D. This comprehensive model aims to allow robots to accurately localize things in a 3D environment based on open natural language queries.
Meta location 3D 3D withdrawals directly from RGB-D sensors (such as those on some robots or sensor cameras on depth). Looking at a text router, such as “flower vase near the TV control unit”, the regime looks at spatial relations and context to determine the right object, and distinguish it, for example, “vase on the table.”
The system includes three main parts: a pre -processing step to convert 2D features into 3D clouds; 3D Jepa encryption (a model before creating a three -dimensional representation of the 3D context); And the site of 3D decoding, which takes 3D representation and linguistic inquiry to remove funds and masks around the specific organisms.
Besides the model, Meta releases a large new data collection to localize the objects based on referral expressions. 130,000 language comments include 1,346 viewers of Arkitscenes, SCANNET and SCANNET ++ data collections, which effectively doubles explained data in this field.
Meta believes that this technology is crucial to developing more capable automatic systems, including its Partnr Robot project, allowing more human natural interaction and cooperation.
Dynamic lytee transformer: effective and powerful language modeling
After research published in late 2024, Meta is now releasing the model for its 8 -billion dynamic bital house.
This structure is a shift away from traditional language models based on the distinctive symbol, and instead works at the home level. Meta claims that this approach is achieving a similar performance widely while providing significant improvements in the efficiency of reasoning and durability.
Traditional LLMS divides the text into “symbols”, which can wrestle with spelling errors, new words, or hostile inputs. Models at home level processing raw households, and may provide greater flexibility.
Meta notes that the BYTE LETET Transformer “is outperforming the distinctive symbol models across various tasks, with a medium durability feature of +7 points (on troubled hellaswag), and reaching up to +55 points in the tasks of the cute distinctive code standards.”
By launching weights alongside the previously shared blade base, Meta encourages the research community to explore this alternative approach to language modeling.
Cooperative reason: Meta is progressing
The final version, the cooperative Distresser, addresses the complex challenge of creating artificial intelligence agents who can effectively cooperate with humans or other artificial intelligence.
Meta notes that human cooperation often gives superior results, and aims to involve artificial intelligence with similar capabilities for tasks such as assistance in home duty or preparing a job interview.
This cooperation requires not only the solution to problems, but also requires social skills such as communication, sympathy, providing comments and understanding mental situations for others (the theory of saying), and often reveals multiple conversation turns.
The current LLM training and evaluation methods often neglect these social and cooperative aspects. Moreover, the collection of relevant conversation data is expensive and difficult.
Distresser cooperative provides a framework for assessing and enhancing these skills. It includes tasks directed towards goals that require multiple -step thinking achieved through conversation between two agents. The frame tests capabilities such as constructive difference, persuading the partner, and reaching a better common solution.
Meta’s reviews revealed that current models are fighting to constantly take advantage of cooperation to get better results. To process this, they suggest the technique of self -improvement using artificial reaction data where the LLM agent cooperates with himself.
The creation of this data is widely enabled by a new high -performance service engine called Matrix. Using this approach to mathematics, reports reported that the tasks of scientific and social thinking resulted in improvements up to 29.4 % compared to the performance of the standard “idea series” for one LLM.
Through the open outbreak of data generation and modeling modeling, Meta aims to enhance more research to create “social agents who can partnership with humans and other agents.”
These five versions that collectively confirm the continuation of heavy investment in Meta in basic artificial intelligence research, with a special focus on the blocks of building machines that can imagine, understand and interact with the world in more human -like ways.
See also: Meta will train artificial intelligence models using European Union user data
Do you want to learn more about artificial intelligence and large data from industry leaders? Check the AI and Big Data exhibition in Amsterdam, California and London. The comprehensive event was identified with other leading events including the smart automation conference, Blockx, the digital transformation week, and the Cyber Security & Cloud.
Explore the upcoming web events and seminars with which Techforge works here.
Don’t miss more hot News like this! Click here to discover the latest in AI news!
2025-04-17 16:00:00