[2502.06124] Foundation Model of Electronic Medical Records for Adaptive Risk Estimation
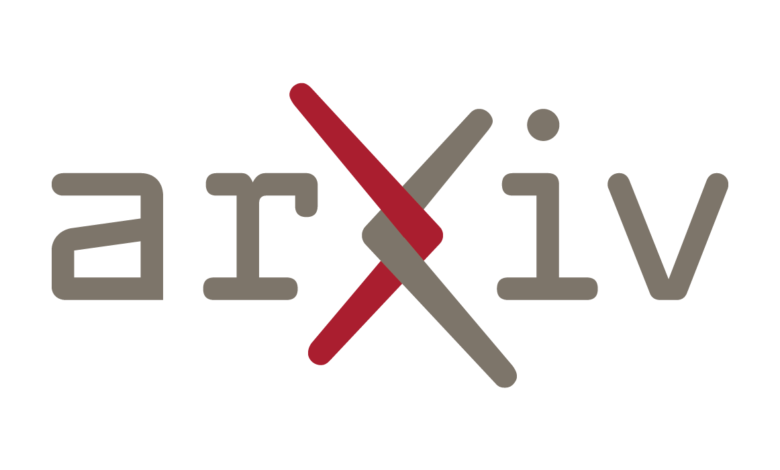
View the PDF file for the paper entitled The Foundation Form of Electronic Medical Records for Adaptive Risks, by Pawel Renc and 10 other authors
PDF HTML (experimental) view
a summary:The United States dedicates approximately 18 % of its gross domestic product for health care, but it suffers from a decrease in average life and high mortality that can be prevented compared to other high income countries. Hospitals are struggled to predict critical results such as deaths, acceptance of the intensive care unit, and long hospital residences. Traditional early warning systems, such as news and MEW, rely on fixed variables and fixed thresholds, which limits adaptation, accuracy and customization. We have developed the improved transformer to simulate health results (ETHOS), a model of artificial intelligence that earns the EHRS health timetables (PHTS) and uses transformers -based structures to predict future decorations. ARS risk estimation system (ARES) works to benefit from ethics to calculate the possibilities of dynamic and personal risks of critical events known by doctors. ARS is also characterized by a customized explanation unit that highlights the main clinical factors that affect risk estimates. We evaluated ARS on the Mimic-IV V2.2 data set in the emergency department settings, and put its performance against traditional early warning systems and automated learning models. From 299,721 unique patients, 285,622 degrees (60 % with hospital acceptance), which includes more than 357 million icons. Ethos outperformed the standard models in the prediction of the acceptance of hospitals, the acceptance of the intensive care unit, the long residencies, and the achievement of superior AUC degrees. Risk estimates were strong through demographic sub -groups, with calibration curves that confirm the reliability of the model. The Clarification Unit provided valuable visions in the patient’s danger factors. ARES, supported by ethics, healthcare provides artificial intelligence by providing a dynamic estimate, in actual time, and personal with the ability of the patient’s explanation. It provides adaptation and accuracy of a transformative tool for clinical decisions, which may improve the patient’s results and customize resources.
The application date
From: Powell Rink [view email]
[v1]
Monday, 10 February 2025 03:22:39 UTC (3,715 KB)
[v2]
Saturday, 8 Mar 2025 18:48:54 UTC (3,715 KB)
[v3]
Thursday, 13 Mar 2025 22:37:55 UTC (3,715 KB)
2025-03-17 04:00:00