Optimal transport for generating transition states in chemical reactions
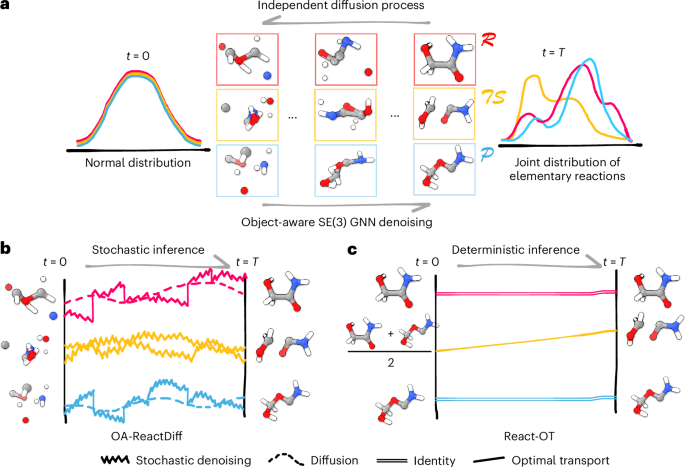
Truhlar, D. G., Garrett, B. C. & Klippenstein, S. J. Current status of transition-state theory. J. Phys. Chem. 100, 12771–12800 (1996).
Google Scholar
E, W. & Vanden-Eijnden, E. Transition-path theory and path-finding algorithms for the study of rare events. Annu. Rev. Phys. Chem. 61, 391–420 (2010).
Google Scholar
Dewyer, A. L., Argüelles, A. J. & Zimmerman, P. M. Methods for exploring reaction space in molecular systems. WIREs Comput. Mol. Sci. 8, e1354 (2018).
Google Scholar
Unsleber, J. P. & Reiher, M. The exploration of chemical reaction networks. Annu. Rev. Phys. Chem. 71, 121–142 (2020). PMID: 32105566.
Google Scholar
Klucznik, T. et al. Computational prediction of complex cationic rearrangement outcomes. Nature 625, 508–515 (2024).
Back, S. et al. Accelerated chemical science with AI. Digit. Discov. 3, 23–33 (2024).
Google Scholar
Nandy, A. et al. Computational discovery of transition-metal complexes: from high-throughput screening to machine learning. Chem. Rev. 121, 9927–10000 (2021).
Google Scholar
Zhang, S. et al. Exploring the frontiers of condensed-phase chemistry with a general reactive machine learning potential. Nat. Chem. https://doi.org/10.1038/s41557-023-01427-3 (2024).
Prozument, K. et al. Photodissociation transition states characterized by chirped pulse millimeter wave spectroscopy. Proc. Natl Acad. Sci. USA 117, 146–151 (2020).
Google Scholar
Liu, Y. et al. Rehybridization dynamics into the pericyclic minimum of an electrocyclic reaction imaged in real-time. Nat. Commun. 14, 2795 (2023).
Google Scholar
Mardirossian, N. & Head-Gordon, M. Thirty years of density functional theory in computational chemistry: an overview and extensive assessment of 200 density functionals. Mol. Phys. 115, 2315–2372 (2017).
Google Scholar
Weinan, E., Ren, W. & Vanden-Eijnden, E. String method for the study of rare events. Phys. Rev. B 66, 052301 (2002).
Google Scholar
Peters, B., Heyden, A., Bell, A. T. & Chakraborty, A. A growing string method for determining transition states: comparison to the nudged elastic band and string methods. J. Chem. Phys. 120, 7877–7886 (2004).
Google Scholar
Sheppard, D., Terrell, R. & Henkelman, G. Optimization methods for finding minimum energy paths. J. Chem. Phys. 128, 134106 (2008).
Google Scholar
Maeda, S., Taketsugu, T. & Morokuma, K. Exploring transition state structures for intramolecular pathways by the artificial force induced reaction method. J. Comput. Chem. 35, 166–173 (2014).
Google Scholar
Shang, C. & Liu, Z. P. Stochastic surface walking method for structure prediction and pathway searching. J. Chem. Theory Comput. 9, 1838–1845 (2013).
Google Scholar
Durant, J. L. Evaluation of transition state properties by density functional theory. Chem. Phys. Lett. 256, 595–602 (1996).
Google Scholar
Zimmerman, P. M. Automated discovery of chemically reasonable elementary reaction steps. J. Comput. Chem. 34, 1385–1392 (2013).
Google Scholar
Simm, G. N., Vaucher, A. C. & Reiher, M. Exploration of reaction pathways and chemical transformation networks. J. Phys. Chem. A 123, 385–399 (2019).
Google Scholar
Unsleber, J. P. et al. High-throughput ab initio reaction mechanism exploration in the cloud with automated multi-reference validation. J. Phys. Chem. 158, 084803 (2023).
Google Scholar
Zhao, Q. & Savoie, B. M. Simultaneously improving reaction coverage and computational cost in automated reaction prediction tasks. Nat. Comput. Sci. 1, 479–490 (2021).
Google Scholar
Yuan, E. C.-Y. et al. Analytical ab initio Hessian from a deep learning potential for transition state optimization. Nat. Commun. 15, 8865 (2024).
Google Scholar
Wang, L.-P. et al. Discovering chemistry with an ab initio nanoreactor. Nat. Chem. 6, 1044–1048 (2014).
Google Scholar
Pieri, E. et al. The non-adiabatic nanoreactor: towards the automated discovery of photochemistry. Chem. Sci. 12, 7294–7307 (2021).
Google Scholar
Zeng, J., Cao, L., Xu, M., Zhu, T. & Zhang, J. Z. H. Complex reaction processes in combustion unraveled by neural network-based molecular dynamics simulation. Nat. Commun. 11, 5713 (2020).
Google Scholar
Van de Vijver, R. & Zádor, J. Kinbot: automated stationary point search on potential energy surfaces. Comput. Phys. Commun. 248, 106947 (2020).
Google Scholar
von Lilienfeld, O. A., Müller, K.-R. & Tkatchenko, A. Exploring chemical compound space with quantum-based machine learning. Nat. Rev. Chem. 4, 347–358 (2020).
Google Scholar
Margraf, J. T., Jung, H., Scheurer, C. & Reuter, K. Exploring catalytic reaction networks with machine learning. Nat. Catal. 6, 112–121 (2023).
Google Scholar
Schreiner, M., Bhowmik, A., Vegge, T., Jørgensen, P. B. & Winther, O. NeuralNEB—neural networks can find reaction paths fast. Mach. Learn. Sci. Technol. 3, 045022 (2022).
Google Scholar
Zhang, S. et al. Exploring the frontiers of condensed-phase chemistry with a general reactive machine learning potential. Nat. Chem. 16, 727–734 (2024).
Google Scholar
Zhang, J. et al. Deep reinforcement learning of transition states. Phys. Chem. Chem. Phys. 23, 6888–6895 (2021).
Google Scholar
Holdijk, L. et al. Stochastic optimal control for collective variable free sampling of molecular transition paths. Adv. Neural Inf. Process. Syst. 36, 79540–79556 (2023).
Pattanaik, L., Ingraham, J. B., Grambow, C. A. & Green, W. H. Generating transition states of isomerization reactions with deep learning. Phys. Chem. Chem. Phys. 22, 23618–23626 (2020).
Google Scholar
van Gerwen, P. et al. EquiReact: an equivariant neural network for chemical reactions. Preprint at https://arxiv.org/abs/2312.08307v2 (2023).
Makoś, M. Z., Verma, N., Larson, E. C., Freindorf, M. & Kraka, E. Generative adversarial networks for transition state geometry prediction. J. Chem. Phys. 155, 024116 (2021).
Google Scholar
Choi, S. Prediction of transition state structures of gas-phase chemical reactions via machine learning. Nat. Commun. 14, 1168 (2023).
Google Scholar
Ho, J., Jain, A. & Abbeel, P. in Advances in Neural Information Processing Systems (eds Larochelle, H. et al.) vol. 33, 6840–6851 (Curran Associates, 2020).
Kim, S., Woo, J. & Kim, W. Y. Diffusion-based generative AI for exploring transition states from 2D molecular graphs. Nat. Commun. 15, 341 (2024).
Google Scholar
Duan, C., Du, Y., Jia, H. & Kulik, H. J. Accurate transition state generation with an object-aware equivariant elementary reaction diffusion model. Nat. Comput. Sci. 3, 1045–1055 (2023).
Google Scholar
Cheng, A. H., Lo, A., Miret, S., Pate, B. H. & Aspuru-Guzik, A. Determining 3D structure from molecular formula and isotopologue rotational spectra in natural abundance with reflection-equivariant diffusion. J. Chem. Phys. 160, 124115 (2024).
Google Scholar
Duan, C., Nandy, A., Meyer, R., Arunachalam, N. & Kulik, H. J. A transferable recommender approach for selecting the best density functional approximations in chemical discovery. Nat. Comput. Sci. 3, 38–47 (2023).
Google Scholar
Corso, G., Stärk, H., Jing, B., Barzilay, R. & Jaakkola, T. DiffDock: diffusion steps, twists, and turns for molecular docking. Preprint at https://arxiv.org/abs/2210.01776 (2023).
Zhao, Q. et al. Comprehensive exploration of graphically defined reaction spaces. Sci. Data 10, 145 (2023).
Google Scholar
Bannwarth, C., Ehlert, S. & Grimme, S. GFN2-xTB—an accurate and broadly parametrized self-consistent tight-binding quantum chemical method with multipole electrostatics and density-dependent dispersion contributions. J. Chem. Theory Comput. 15, 1652–1671 (2019).
Google Scholar
Sohl-Dickstein, J., Weiss, E., Maheswaranathan, N. & Ganguli, S. Deep unsupervised learning using nonequilibrium thermodynamics. In International Conference on Machine Learning, PMLR 37, 2256–2265 (2015).
Song, Y. et al. Score-based generative modeling through stochastic differential equations. In International Conference on Learning Representations. Preprint at https://arxiv.org/abs/2011.13456v2 (2021).
Lipman, Y., Chen, R. T. Q., Ben-Hamu, H., Nickel, M. & Le, M. Flow matching for generative modeling. In The Eleventh International Conference on Learning Representations (ICLR, 2023).
Liu, G.-H. et al. I2SB: Image-to-image Schrödinger bridge. In International Conference on Machine Learning (ICLR, 2023).
Somnath, V. R. et al. Aligned diffusion Schrödinger bridges. In Proc. 39th Conference on Uncertainty in Artificial Intelligence. Vol 216 (PMLR, 2023).
Zhao, Q., Hsu, H.-H. & Savoie, B. Conformational sampling for transition state searches on a computational budget. J. Chem. Theory Comput. 18, 3006–3016 (2022).
Google Scholar
Sindhu, A., Pradhan, R., Lourderaj, U. & Paranjothy, M. Theoretical investigation of the isomerization pathways of diazenes: torsion vs. inversion. Phys. Chem. Chem. Phys. 21, 15678–15685 (2019).
Google Scholar
Koda, S.-i & Saito, S. Locating transition states by variational reaction path optimization with an energy-derivative-free objective function. J. Chem. Theory Comput. 20, 2798–2811 (2024).
Google Scholar
Schreiner, M., Bhowmik, A., Vegge, T., Busk, J. & Winther, O. Transition1x—a dataset for building generalizable reactive machine learning potentials. Sci. Data 9, 779 (2022).
Google Scholar
Henkelman, G., Uberuaga, B. P. & Jónsson, H. A climbing image nudged elastic band method for finding saddle points and minimum energy paths. J. Phys. Chem. 113, 9901–9904 (2000).
Google Scholar
Chai, J.-D. & Head-Gordon, M. Systematic optimization of long-range corrected hybrid density functionals. J. Phys. Chem. 128, 084106 (2008).
Google Scholar
Ditchfield, R., Hehre, W. J. & Pople, J. A. Self-consistent molecular-orbital methods. IX. an extended Gaussian-type basis for molecular-orbital studies of organic molecules. J. Phys. Chem. 54, 724–728 (1971).
Google Scholar
Grambow, C. A., Pattanaik, L. & Green, W. H. Reactants, products, and transition states of elementary chemical reactions based on quantum chemistry. Sci. Data 7, 137 (2020).
Google Scholar
Grambow, C. A., Pattanaik, L. & Green, W. H. Deep learning of activation energies. J. Phys. Chem. Lett. 11, 2992–2997 (2020).
Google Scholar
Ruddigkeit, L., van Deursen, R., Blum, L. C. & Reymond, J.-L. Enumeration of 166 billion organic small molecules in the chemical universe database GDB-17. J. Chem. Inf. Model. 52, 2864–2875 (2012).
Google Scholar
Du, W. et al. A new perspective on building efficient and expressive 3D equivariant graph neural networks. Adv. Neural Inf. Process. Syst. 36, 66647–66674 (2023).
Fu, H., Zhou, Y., Jing, X., Shao, X. & Cai, W. Meta-analysis reveals that absolute binding free-energy calculations approach chemical accuracy. J. Med. Chem. 65, 12970–12978 (2022).
Google Scholar
Bremond, E., Li, H., Perez-Jimenez, A. J., Sancho-Garcia, J. C. & Adamo, C. Tackling an accurate description of molecular reactivity with double-hybrid density functionals. J. Chem. Phys. 156, 161101 (2022).
Google Scholar
Zhao, Q. & Savoie, B. M. Algorithmic explorations of unimolecular and bimolecular reaction spaces. Angew. Chem. Int. Ed. 61, e202210693 (2022).
Google Scholar
Grambow, C. A. et al. Unimolecular reaction pathways of a γ-ketohydroperoxide from combined application of automated reaction discovery methods. J. Am. Chem. Soc. 140, 1035–1048 (2018).
Google Scholar
Naz, E. G. & Paranjothy, M. Unimolecular dissociation of γ-ketohydroperoxide via direct chemical dynamics simulations. J Phys. Chem. A 124, 8120–8127 (2020).
Google Scholar
Ramakrishnan, R., Dral, P. O., Rupp, M. & von Lilienfeld, O. A. Quantum chemistry structures and properties of 134 kilo molecules. Sci. Data 1, 140022 (2014).
Google Scholar
Jain, A. et al. Commentary: The Materials Project: a materials genome approach to accelerating materials innovation. APL Mater. 1, 011002 (2013).
Google Scholar
Tran, R. et al. The Open Catalyst 2022 (OC22) dataset and challenges for oxide electrocatalysts. ACS Catal. 13, 3066–3084 (2023).
Google Scholar
Chmiela, S. et al. Machine learning of accurate energy-conserving molecular force fields. Sci. Adv. 3, e1603015 (2017).
Google Scholar
Chmiela, S. et al. Accurate global machine learning force fields for molecules with hundreds of atoms. Sci. Adv. 9, eadf0873 (2023).
Google Scholar
Nandi, S., Vegge, T. & Bhowmik, A. MultiXC-QM9: large dataset of molecular and reaction energies from multi-level quantum chemical methods. Sci. Data 10, 783 (2023).
Google Scholar
Birkholz, A. B. & Schlegel, H. B. Using bonding to guide transition state optimization. J. Comput. Chem. 36, 1157–1166 (2015).
Google Scholar
Kovács, D. P. et al MACE-OFF: Transferable machine learning force fields for organic molecules. Preprint at https://arxiv.org/abs/2312.15211 (2023).
Zhang, D., Liu, X. & Zhang, X. DPA-2: a large atomic model as a multi-task learner. NPJ Comput. Mater 10, 293 (2024).
Google Scholar
Du, Y. et al. in Advances in Neural Information Processing Systems, (eds Oh, A. et al.) vol. 36, 77359–77378 (Curran Associates, 2023).
Du, Y. et al. Doob’s Lagrangian: a sample-efficient variational approach to transition path sampling. In (Globerson. A. et al. eds) Advances in Neural Information Processing Systems 37, 65791–65822 (2024).
Serre, J.-P. et al. Linear Representations of Finite Groups vol. 42 (Springer, 1977).
Bronstein, M. M., Bruna, J., Cohen, T. & Veličković, P. Geometric deep learning: grids, groups, graphs, geodesics, and gauges. Preprint at https://arxiv.org/abs/2104.13478 (2021).
Köhler, J., Klein, L. & Noé, F. Equivariant flows: exact likelihood generative learning for symmetric densities. In International Conference on Machine Learning 5361–5370 (2020).
Villani, C. et al. Optimal Transport: Old and New vol. 338 (Springer, 2009).
Villani, C. Topics in Optimal Transportation vol. 58 (American Mathematical Society, 2021).
Santambrogio, F. Optimal transport for applied mathematicians. Birkäuser 55, 94 (2015).
Zhang, L. & Wang, L. Monge-amp\ere flow for generative modeling. Preprint at https://arxiv.org/abs/1809.10188 (2018).
Monge, G. Mémoire sur la théorie des déblais et des remblais. Imprimerie Royale (1781).
Kantorovich, L. V. On the translocation of masses. Dokl. Akad. Nauk. 37, 199–201 (1942).
Benamou, J.-D. & Brenier, Y. A computational fluid mechanics solution to the Monge–Kantorovich mass transfer problem. Num. Math. 84, 375–393 (2000).
Google Scholar
Peyré, G. & Cuturi, M. Computational Optimal Transport (Center for Research in Economics and Statistics Working Papers, 2017).
Liu, X., Gong, C. & Liu, Q. Flow straight and fast: learning to generate and transfer data with rectified flow. In 11th International Conference on Learning Representations (2023).
Shen, Z. et al. Accurate point cloud registration with robust optimal transport. Adv. Neural Inf. Process. Syst. 34, 5373–5389 (2021).
Titouan, V., Courty, N., Tavenard, R. & Flamary, R. Optimal transport for structured data with application on graphs. In International Conference on Machine Learning 6275–6284 (PMLR, 2019).
Lugmayr, A. et al. Repaint: inpainting using denoising diffusion probabilistic models. In 2022 IEEE/CVF Conference on Computer Vision and Pattern Recognition (2022).
Schreiner, M. et al. Transition1x. Figshare https://doi.org/10.6084/m9.figshare.19614657.v4 (2022).
Zhao, Q. et al. Reaction dataset. Zenodo https://doi.org/10.5281/zenodo.13119868 (2024).
Zhao, Q. deepprinciple/react-ot: reactot. Zenodo https://doi.org/10.5281/zenodo.14836384 (2025).
Don’t miss more hot News like this! Click here to discover the latest in AI news!
2025-04-23 00:00:00