SWiRL: The business case for AI that thinks like your best problem-solvers
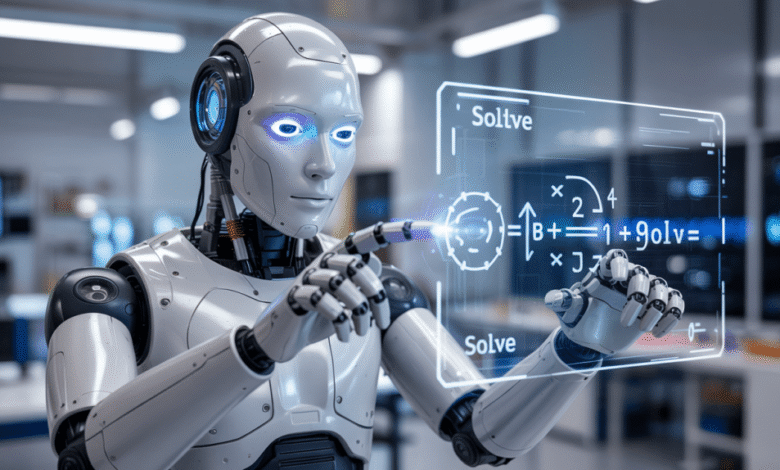
Join daily and weekly newsletters to obtain the latest updates and exclusive content to cover the leading artificial intelligence in the industry. Learn more
Researchers from Stanford University and Google DeepMind have revealed step -by -step reinforcement learning (SWIRL), a technique designed to enhance the ability of large LLMS models to address complex tasks that require multiple -step thinking and use of tools.
With the continued increase in interest in artificial intelligence factors and the LLM tool, this technology can provide great advantages for institutions that look forward to integrating thinking models in their applications and workflow.
Challenge multi -steps
The real world’s applications often include multi -step processes. For example, planning for a complex marketing campaign may include market research, internal data analysis, budget account and review customer support tickets. This requires online searches, access to internal databases and symbol.
Traditional traditional learning methods (RL) (RL) focus to adjust LLMS, such as the reinforcement learning from human comments (RLHF) or RL of AI (RLAIF), usually focuses on improving models for a single -step thinking tasks.
The main authors of a spiral paper, Anna Goldi, a research scientist Google DeepMind, and Azalia Mirhosseini, a computer science aid at Stanford University, believe that current LLM training methods are not suitable for multi -step thinking tasks required by real world applications.
“LLMS trained through traditional methods usually struggle with multi -step planning and integration of tools, which means that they are having difficulty performing tasks that require documents recovery and synthesis from multiple sources (for example, writing a work report) or multiple steps of thinking and account account (for example, preparing a financial summary).”
Learn step -by -step reinforcement (vortex)
The vortex of this multi -step challenge is dealt with a set of artificial data generation and a specialized RL approach that trains models on a full sequence of procedures.
The researchers also state in their paper, “Our goal is to teach the model how to decompose complex problems in a series of more managed sub -tasks, when the tool can be called, how to formulate an invitation to the tool, and when the results of these queries are used to answer the question, and how to manufacture its results effectively.”
It uses a systematic cycle in two phases. First, it creates and filters large quantities of multi -step thinking data and using tools. Second, the RL algorithm, which is step -by -step, uses LLM to improve the base using these created paths.
“This approach has a major practical feature that we can create quickly large quantities of multi -step training data through parallel calls to avoid suffocation of the training process by implementing the slow tool,” notes paper. “In addition, this incompatible process allows greater cloning due to the presence of a fixed data set.”
Training data generation
The first stage includes the creation of an artificial data spiral from which you learn. LLM is given access to a relevant tool, such as search engine or calculator. Then the model is required to repeat the creation of a “path”, which is a series of steps to solve a specific problem. In each step, the model can generate internal thinking (“” Thought “), call a tool, or produce the final answer. If a tool is required, the query is extracted and executed (for example, a research is made), and the result is fed again in the context of the model for the next step. This continues until the model provides a final answer.
Then each entire path, from the initial demand to the final answer, is divided into many overlapping sub -tracks. Each sub -path represents the process until a specific procedure, which provides a likable performance of the logic with a step -by -step. Using this method, the team collected large data sets based on questions from the multiple answer criteria for the rules (Hotpotqa) and problem solving standards (GSM8K), generating tens of thousands of tracks.
Researchers explore four different data filtering strategies: there is no liquidation, liquidation based only on the validity of the final answer (liquidation of results), liquidation based on the reasonableness shown for each individual step (liquidation of the process) and liquidation based on both the process and the result.
Many standard methods, such as the SFT control, depends greatly on the “golden stickers” (the perfect correct answers, and often ignore data that does not lead to the correct final answer. Modern RL methods are also used, such as those used in Deepseek-R1, the results based on results to train the model.
On the other hand, the whirlpool has achieved its best results using the filter data for the operation. This means that the data includes paths where each step is considered a step or the tool is logical in view of the previous context, even if the final answer turns into an error.
The researchers have found that the vortex can “can even learn from the paths that end in the incorrect final answers. In fact, we achieve our best results by including the nominated data, regardless of the validity of the result.”
LLMS training with a spiral
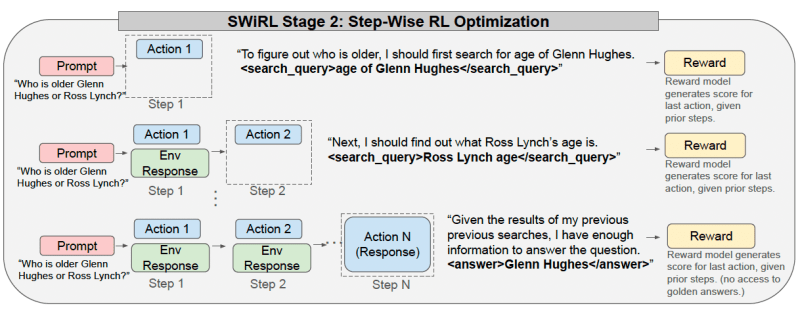
In the second stage, the reinforcement learning cycle is used for the basic LLM training on the created artificial paths. In each step within a path, the model is improved to predict the following appropriate procedure (medieval thinking step, tool call or final answer) based on the previous context.
LLM receives notes at each step by a separate obstetric bonus model, which establishes the creature of the model in view of the context to that point.
“Our granular model, step by step, allows the model to learn both local decisions (prediction the next step) and improve the global path (the generation of the final response) while directing it through immediate reactions to the integrity of each prediction,” the researchers write.
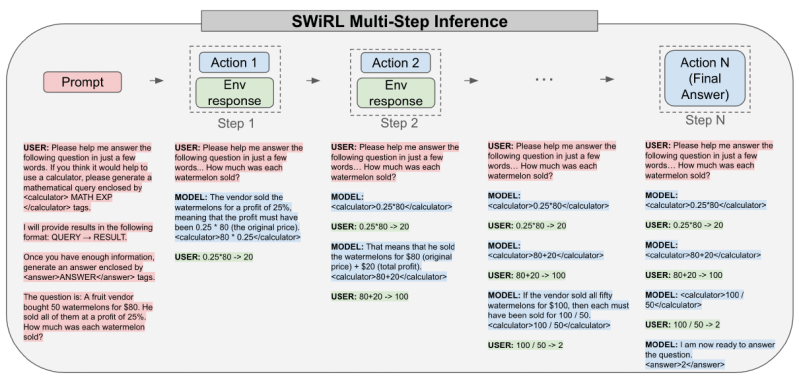
At the time of reasoning, the coach of the vortex operates with the same repetitive style. It receives a claim and creates the text in the response. If he takes out a tool call (such as research query or sporting expression), the system expands it, executes the tool, nourishes the result again in the window window. Then the model continues to generate more tool calls, until it takes out a final answer or reaches a predetermined extent to the number of steps.
“By training the model to take reasonable steps at every moment (and to do this in a coherent and more interpretation in a), we are essentially weakened in the traditional LLMS, which is their fragility in the face of complex multi -step tasks, as it determines the possibility of success significantly with the length of the path,” said Goldi and Mirin. “The useful and powerful AI will need to merge a wide range of different tools, and its sequence together in complex sequences.”
A spiral at work
The Stanford and Google DeepMind team evaluated the vortex through many multi -step -to -step answers tasks. Compared to the basic models, the vortex of significant improvements in the relative accuracy, which ranges from 11 % to more than 21 % on data groups such as GSM8K, Hotpotqa, Musique and Beerqa.
Experiments have confirmed that the GMMA 2-27B model training with a spiral on the data that has been classified on the process has resulted in the best results, or outperformed models that have been trained in data provided by the results or using traditional SFT. This indicates that a whirlpool learns the basic thinking process more effectively, instead of just saving paths to correct answers, which help performance on invisible problems.
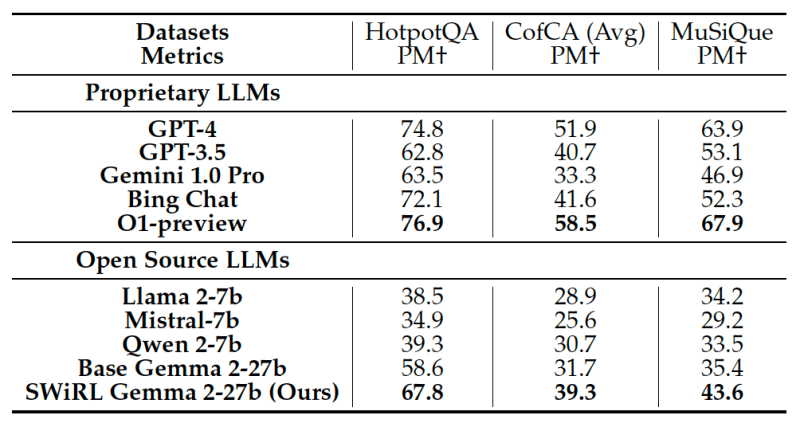
More importantly, the cycle of strong generalization capabilities showed. For example, he trained a model using a spiral on examples to cancel questions based on the text on its performance of mathematics thinking tasks, although the model was not explicitly trained in mathematics problems.
This transfer ability through tasks and different types of tools is of high value because there is an explosion for the agent applications of language models, and the methods that are generalized through data collections and tasks will be easier, cheaper and faster to adapt to new environments.
“The generalization of a spiral is very strong in the areas we discovered, but it will be interesting to test this in other areas such as coding,” said Goldi and Mersheny. “The results we have found indicate that the AI model of the trained institution is one of the basic tasks that use a vortex that is likely to show great performance improvements on other tasks, which seems unrelated without this technology being more effective in future capabilities when it grows in larger models (i.e. more powerful), indicating that this technique may be more effective in future capabilities.”
Don’t miss more hot News like this! Click here to discover the latest in AI news!
2025-04-22 23:53:00