When AI reasoning goes wrong: Microsoft Research shows more tokens can mean more problems
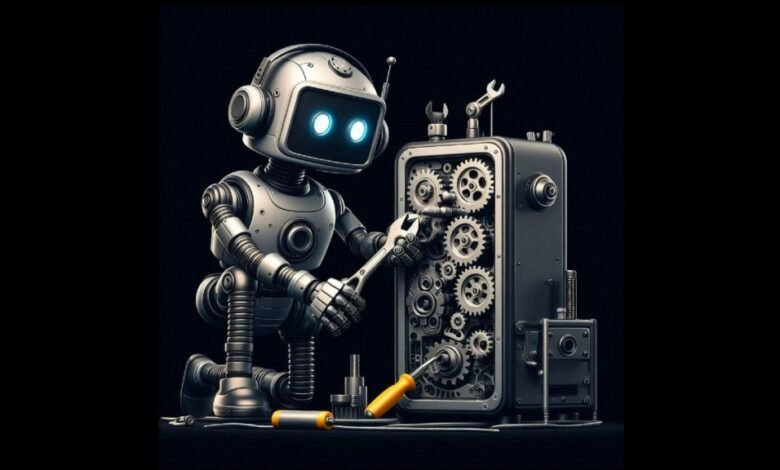
Join daily and weekly newsletters to obtain the latest updates and exclusive content to cover the leading artificial intelligence in the industry. Learn more
LLMS models are increasingly capable of complex thinking by “limiting the time of reasoning”, a group of technologies that allocate more arithmetic resources during reasoning to create answers. However, a new study of Microsoft Research reveals that these scaling methods are not universal. Performance reinforcements vary greatly through different models, tasks and complications.
The basic conclusion is that just throwing more account in a problem during reasoning does not guarantee better or more efficient results. The results can help in better understanding institutions to volatility and reliability of the model because they look forward to integrating advanced thinking in artificial intelligence applications in their applications.
Setting scaling methods on the test
The Microsoft Research team conducted a wide experimental analysis across nine modern institution models. This included both “traditional” models such as GPT-4O, Claude 3.5 Sonnet, Gemini 2.0 Pro and Llama 3.1 405b, as well as models that were specifically seized to enhance thinking by scaling at a reasoning time. This included Openai’s O1 and O3-MINI, Claude 3.7 Sonnet, and Deepseek R1.
They evaluated these models using three outstanding approaches for a distinct conclusion:
- Standard Idea series: The main method where the model is required to answer step by step.
- Parallel scaling: The model generates multiple independent answers to the same question and uses a complex (such as the majority vote or the best better answer) to reach a final result.
- Serial expansion: The model generates a frequency of an answer and uses reactions from the critic (it is likely to be the same model) to improve the answer in subsequent attempts.
These methods have been tested on eight difficult data sets challenge covering a wide range of tasks that benefit from problem-step solution: thinking about mathematics and continuity (AIME, OMNI-Math, GPQA), calendar layout, NP-Hard Besinches (3Sat, TSP), Navingng (MAZE) (Maze).
Many criteria included problems with varying difficulty, which allows more accurate understanding of how to behave with problems with problems.
“The availability of difficulty signs for OMNI-Math, TSP, 3Sat and Ba-Calendar enables us to analyze the accuracy of use and distinctive symbol with difficulty in limiting the time of reasoning, which is still an unstable perspective,” the researchers wrote in the paper that explains their results.
The researchers evaluated the Barito LLM border by analyzing both accuracy and mathematical cost (i.e. the number of symbols created). This helps to determine how the models are efficiently achieving their results.

They also provided the “traditional gap to the gap”, which compares the best possible performance of the traditional model (using a better choice of “the ideal n) for the average performance of the thinking model, which appreciates the potential gains that can be achieved through better training or verification techniques.
More account is not always the answer
The study presented many decisive ideas that challenge common assumptions about limiting the time of reasoning:
Benefits vary greatly: Although the models that were seized for thinking generally outperform the traditional models in these tasks, the degree of improvement varies greatly depending on the field and the specified task. The gains often diminish with increased complexity of the problem. For example, performance improvements in mathematics problems have not always been translated evenly into scientific thinking tasks or planning.
Avoid efficiency is widespread: The researchers have noticed a great contrast in the consumption of the distinctive symbol, even among models that achieve similar accuracy. For example, in AIME 2025 Math Math, use Deepseek-R1 more than five times the distinctive symbols more than Claude 3.7 Sonnet for almost similar medium accuracy.
More symbols do not lead to a higher accuracy: Unlike the intuitive idea that longer thinking chains mean better thinking, the study found that this is not always true. “Surprisingly, we also note that the longest generations for the model itself can sometimes be an indication of the struggling models, rather than improving thinking,” says the paper. “Likewise, when comparing different thinking models, the use of the most distinctive symbol is not always associated with better accuracy. These results stimulate the need for more quieter and cost -effective scaling methods.”
Nonderminism cost: Perhaps what is most important to the institution’s users, frequent queries can lead to the same form of the same problem to the use of a very distinctive symbol. This means that the cost of running the query can volatility, even when the model constantly provides the correct answer.

The capabilities in verification mechanisms: The scaling performance is constantly improved in all models and standards when simulating “perfect verification” (using the best N results).
Traditional models are sometimes identical to thinking models: By significantly increasing the inference of the reasoning (up to 50x more in some experiments), traditional models such as GPT-4O can sometimes come close to the levels of performing dedicated thinking models, especially in less complex tasks. However, these gains quickly reduced in highly complex settings, indicating that the limitation of brute force has its limits.

The effects of the institution
These results carry a great weight for the developers and institutions adopted from LLMS. The issue of “unspecified cost” in particular and makes the budget difficult. The researchers also note, “Ideally, developers and users prefer models that are the standard deviation around the use of the distinctive symbol for each counterpart in the cost.”
“The stereotype we do [the study] It may be useful for developers as a tool for choosing models that are less volatile for the same claim or for different demands. ”Pismera Nushchi, great headmaster of Microsoft Research, Venturebeat.

The study also provides good visions in the relationship between the accuracy of the model and the length of response. For example, the following graph shows that mathematics queries that exceed about 11,000 of the distinctive symbol length have a very small chance of correction, and these generations should be stopped at this point or restarted with some serial comments. However, Nushi notes that models that allow these custom discings after they contain a cleaner separation between the correct and incorrect samples.

“Ultimately, the models of models are also liability to think about reducing accuracy and non -living cost, and we expect a lot of this to happen with increasing maturity,” Nuchi said. “Besides the unspecified cost, the unlimited accuracy applies as well.”
Another important result is the fixed performance of Verfiers Perfect, which highlights an important field for future work: building strong and widely applicable verification mechanisms.
“The availability of the most powerful verification can have different types of influence,” Nuchi said, such as improving basic training methods of thinking. “If it is used efficiently, these can limit the effects of thinking.”
Strong verification can also become a major part of AI Agency solutions. Many stakeholders in the institution already have such selections, which you may need to reuse for more agent solutions, such as Sat Satlaves, logistical validity auditors, etc.
“The future questions are how such current techniques can be combined with AI’s interfaces and what language links the two.” “The necessity of communicating the two comes from the fact that users will not always formulate their inquiries in an official way, they will want to use a natural language interface and expect solutions in a similar coordination or in a final procedure (for example, suggested inviting a meeting).”
Don’t miss more hot News like this! Click here to discover the latest in Technology news!
2025-04-15 23:50:00