Our latest advances in robot dexterity
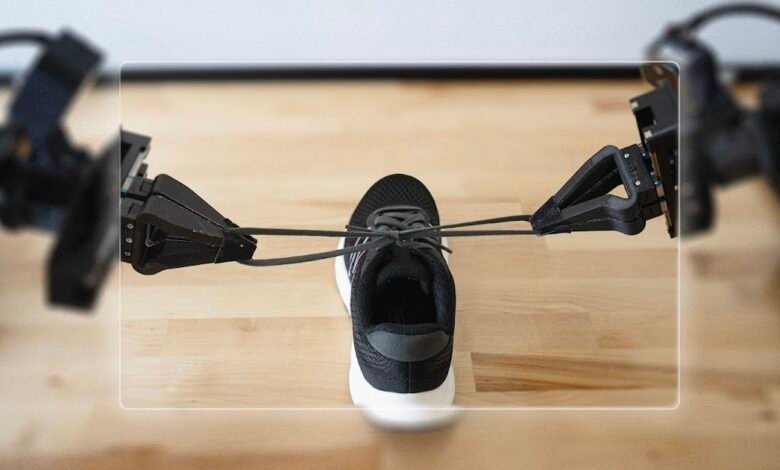
research
Two new artificial intelligence systems, Aloha Unleashed and Demostart, help robots learn to perform complex tasks that require a beautiful movement
People do many tasks on a daily basis, such as connecting the ligaments of the shoe or tightening the screw. But for robots, it is extremely difficult to learn these high -time tasks. To make robots more useful in people’s lives, they need to improve in contact with physical things in dynamic environments.
Today, we offer two new sheets that include the latest progress in artificial intelligence (AI) in the ingenuity research in the robot: I launched its aloe that helps robots learn to perform complex tasks and armed novel; Demostart, which uses simulations to improve performance in the real world by a multi -wicked mechanism.
By helping robots learn from human demonstrations and translating images to work, these systems pave the way for robots that can perform a wide range of useful tasks.
Improve imitation learning with two mechanisms
To date, most of the most advanced artificial intelligence robots have been able to capture and place objects with one arm. In our new paper, we unleash the aloe, which achieves a high level of ingenuity in bipolar manipulation. Through this new method, our robot learns to tie shoes, hang a shirt, fix another robot, insert a gear and even clean the kitchen.
An example of the ligaments of dual -arm robot shoes and tie them in the bow.
An example of a dual -arm robot puts a Polo shirt on a table, put it on the clothes hanger and then hang it on a shelf.
An example of a dual -arm robot repair another robot.
The Aloha Unleashed method depends on our Aloha 2 platform, which was based on the original Aloha system (which is a low -cost open source system for Bimanual Telepertation) from Stanford University.
Aloha 2 is more skillful than the previous systems because it contains my hand that can be easily activated for training and data collection, and robots are allowed to know how to perform new tasks with lower demonstrations.
We have also improved the work environment in robotic devices and we have improved the learning process in our latest system. First, we collected the explanatory show data by running the robot behavior from a distance, and performing difficult tasks such as linking the shoe ligaments and suspended shirts. Next, we have applied a spread method, predicted robot procedures from random noise, similar to how to create our Imagen model. This robot helps to learn from data, so it can perform the same tasks on its own.
Learn automatic behaviors from some simulation demonstrations
Roboty hand control, a complex task, is more complicated with each additional, detailed and sensor finger. In another new sheet, we offer Demostart, which uses the reinforcement learning algorithm to help robots acquire behaviors preserved in simulation. These behaviors are especially useful for complex embodiment, such as multi -wicked hands.
Demostart first learn from easy situations, and over time, learning starts from more difficult situations until you master the best of its best ability. It takes 100x a simulator of less than 100x to learn how to solve an important simulation than usually required when learning from examples in the real world for the same purpose.
The robot has achieved a success rate of more than 98 % on a number of different tasks in simulation, including redirecting cubes in a certain color, tightening walnuts and actions, and arranging tools. In preparing the real world, a 97 % success rate achieved and raised the cube redirect, and 64 % on the component delivery entry mission requires high -fingers coordination and accuracy.
An example of learning the automatic arm to successfully introduce a yellow conductor in simulation (left) and in preparing the real world (right).
An example of the arm of the mechanism of learning to tighten the bolt on the screw in simulation.
We have developed Demostart with Mujoco, open source physics simulator. After mastering a set of tasks in simulating and using standard techniques to reduce the SIM gap, such as the random distribution of the field, our approach was able to transfer nearly from scratch to the material world.
Automated learning in simulation can reduce the cost and time needed to operate actual material experiences. But it is difficult to design this simulation, and moreover, it does not always translate to successfully returning to the real world’s performance. By combining learning reinforcement and learning from some demonstrations, the gradual learning of Demostart automatically generates curricula that blocks the gap from SIM to realistic, which facilitates the transfer of knowledge from simulation to physical robot, and reduce the cost and time required to operate physical experiences.
To enable more advanced robot learning through intense experience, we tested this new approach by the bad guys mechanism, called Dex-Ee, which was developed in cooperation with Shadow Robot.
DEX-Ee Dexterous Robotic Hand Image, developed by Shadow Robot, in cooperation with the Google DeepMind Robotics team: Shadow Robot).
The future of robot ingenuity
Robotics is a unique field of artificial intelligence research that shows the success of our approaches in the real world. For example, a large language model can tell you how to tighten the bolt or tie your shoes, but even if it is embodied in a robot, he will not be able to perform these same tasks.
One day, artificial intelligence robots will help people in all types of tasks at home, workplace and more. Ingenuity research, including effective and public learning methods that we described today will help make this future possible.
We still have a long way before robots can understand and deal with them easily and accurately, but we are making great progress, and all the pioneering innovation is another step in the right direction.
Thanks and appreciation
Dimostart authors: Maria Bawza, Jose Enrique Chen, Valentin Dalibard, Nimrod Gilidi, Roland Havener, Antoine Lorenz, Merlo F. Martins, Jose Moore, Rogerie Petsivit, Docuante Rao, Martins, Martin Redmaleer, Nuri, Nicholas Hess.
Aloha’s authors were launched: Tony Zao, Jonathan Tompson, Danny Drees, Beit Florence, Kamear Gasimibor, Chelsea Vin, Izan Wahid.
2024-09-12 14:00:00